What is the Best Machine Learning Algorithms for Imbalanced Datasets?
In machine learning, imbalanced datasets are those where one class heavily outnumbers the others. This can be due to the nature of the problem or simply because more data is available for one class than the others. Either way, imbalanced datasets can pose a challenge for machine learning algorithms. In this blog post, we’ll take a look at which machine learning algorithms are best suited for imbalanced datasets and why they tend to perform better than others.
For example, in a binary classification problem, if there are 100 observations, and only 10 of them are positive (the rest are negatives), then we say that the dataset is imbalanced. The ratio of positive to negative cases is 1:10.

There are a few reasons why some machine learning algorithms tend to perform better on imbalanced datasets than others. First, certain algorithms are designed to handle imbalanced datasets. Second, some algorithms are more robust to outliers, which can be more common in imbalanced datasets. And third, some algorithms are better able to learn from a limited amount of data, which can be an issue when one class is heavily outnumbered by the others.
Some of the best machine learning algorithms for imbalanced datasets include:
– Support Vector Machines (SVMs),
– Decision Trees,
– Random Forests,
– Naive Bayes Classifiers,
– k-Nearest Neighbors (kNN),
Of these, SVMs tend to be the most popular choice as they are specifically designed to handle imbalanced datasets. SVMs work by finding a hyperplane that maximizes the margin between the two classes. This helps to reduce overfitting and improve generalization. Decision trees and random forests are also popular choices as they are less sensitive to outliers than other algorithms such as linear regression. Naive Bayes classifiers are another good choice as they are able to learn from a limited amount of data. kNN is also a good choice as it is not sensitive to outliers and is able to learn from a limited amount of data. However, it can be computationally intensive for large datasets.
There are two main types of machine learning algorithms: supervised and unsupervised. Supervised algorithms tend to perform better on imbalanced datasets than unsupervised algorithms. In this blog post, we will discuss why this is so and look at some examples.
Supervised Algorithms
Supervised algorithms are those where the target variable is known. In other words, we have training data where the correct answers are already given. The algorithm then learns from this data and is able to generalize to new data. Some examples of supervised algorithms are regression and classification.
Unsupervised Algorithms
Unsupervised algorithms are those where the target variable is not known. With unsupervised algorithms, we only have input data, without any corresponding output labels. The algorithm has to learn from the data itself without any guidance. Some examples of unsupervised algorithms are clustering and dimensionality reduction.
Why Supervised Algorithms Perform Better on Imbalanced Datasets
The reason why supervised algorithms perform better on imbalanced datasets is because they can learn from the training data which cases are more important. With unsupervised algorithms, all data points are treated equally, regardless of whether they are in the minority or majority class.
For example, in a binary classification problem with an imbalanced dataset, let’s say that we want to predict whether a customer will default on their loan payment or not. We have a training dataset of 1000 customers, out of which only 100 (10%) have defaulted on their loan in the past.
If we use a supervised algorithm like logistic regression, the algorithm will learn from the training data that defaulting on a loan is rare (since only 10% of cases in the training data are Positive). This means that it will be more likely to predict correctly that a new customer will not default on their loan (since this is the majority class in the training data).
However, if we use an unsupervised algorithm like k-means clustering, all data points will be treated equally since there is no target variable to guide the algorithm. This means that it might incorrectly cluster together customers who have defaulted on their loans with those who haven’t since there is no guidance provided by a target variable.
Conclusion:
In conclusion, supervised machine learning algorithms tend to perform better on imbalanced datasets than unsupervised machine learning algorithms because they can learn from the training data which cases are more important.
Some machine learning algorithms tend to perform better on highly imbalanced datasets because they are designed to deal with imbalance or because they can learn from both classes simultaneously. If you are working with a highly imbalanced dataset, then you should consider using one of these algorithms.
Thanks for reading!
How are machine learning techniques being used to address unstructured data challenges?
Machine learning techniques are being used to address unstructured data challenges in a number of ways:
- Natural language processing (NLP): NLP algorithms can be used to extract meaningful information from unstructured text data, such as emails, documents, and social media posts. NLP algorithms can be trained to classify text data, identify key terms and concepts, and extract structured data from unstructured text.
- Image recognition: Machine learning algorithms can be used to analyze and classify images, enabling the automatic identification and classification of objects, people, and other elements in images. This can be useful for tasks such as image tagging and search, as well as for applications such as security and surveillance.
- Audio and speech recognition: Machine learning algorithms can be used to analyze and classify audio data, enabling the automatic transcription and translation of spoken language. This can be useful for tasks such as speech-to-text transcription, as well as for applications such as call center automation and language translation.
- Video analysis: Machine learning algorithms can be used to analyze and classify video data, enabling the automatic detection and classification of objects, people, and other elements in video. This can be useful for tasks such as video tagging and search, as well as for applications such as security and surveillance.
Overall, machine learning techniques are being used in a wide range of applications to extract meaningful information from unstructured data, and to enable the automatic classification and analysis of data in a variety of formats.
How is AI and machine learning impacting application development today?
Artificial intelligence (AI) and machine learning are having a significant impact on application development today in a number of ways:
- Enabling new capabilities: AI and machine learning algorithms can be used to enable applications to perform tasks that would be difficult or impossible for humans to do. For example, AI-powered applications can be used to analyze and classify large amounts of data, or to automate complex decision-making processes.
- Improving performance: AI and machine learning algorithms can be used to optimize the performance of applications, making them faster, more efficient, and more accurate. For example, machine learning algorithms can be used to improve the accuracy of predictive models, or to optimize the performance of search algorithms.
- Streamlining development: AI and machine learning algorithms can be used to automate various aspects of application development, such as testing, debugging, and deployment. This can help to streamline the development process and reduce the time and resources needed to build and maintain applications.
- Enhancing user experiences: AI and machine learning algorithms can be used to enhance the user experience of applications, by providing personalized recommendations, recommendations, or by enabling applications to anticipate and respond to the needs and preferences of users.
Overall, AI and machine learning are having a significant impact on application development today, and they are likely to continue to shape the way applications are built and used in the future.
How will advancements in artificial intelligence and machine learning shape the future of work and society?
Advancements in artificial intelligence (AI) and machine learning are likely to shape the future of work and society in a number of ways. Some potential impacts include:
- Automation: AI and machine learning algorithms can be used to automate tasks that are currently performed by humans, such as data entry, customer service, and manufacturing. This could lead to changes in the types of jobs that are available and the skills that are in demand, as well as to increased productivity and efficiency.
- Job displacement: While automation may create new job opportunities, it could also lead to job displacement, particularly for workers in industries that are more susceptible to automation. This could lead to social and economic challenges, including unemployment and income inequality.
- Increased efficiency: AI and machine learning algorithms can be used to optimize and streamline business processes, leading to increased efficiency and productivity. This could lead to economic growth and innovation, and could also help to reduce costs for businesses and consumers.
- Enhanced decision-making: AI and machine learning algorithms can be used to analyze large amounts of data and make more informed and accurate decisions. This could lead to improved outcomes in fields such as healthcare, finance, and education, and could also help to reduce bias and improve fairness.
Overall, the impact of AI and machine learning on the future of work and society is likely to be significant and complex, with both potential benefits and challenges. It will be important to consider and address these impacts as these technologies continue to advance and become more widely adopted.
- [R] How do you search for implementations of Mixture of Expert models that can be trained locally in a laptop or desktop without ultra-high end GPUs?by /u/Furiousguy79 (Machine Learning) on July 25, 2024 at 11:12 pm
Hi, I am a 2nd year PhD student in CS. My supervisor just got this idea about MoEs and fairness and asked me to implement it ( work on a toy classification problem on tabular data and NOT language data). However as it is not their area of expertise, they did not give any guidelines on how to approach it. My main question is: How do I search for or proceed with implementing a mixture of expert models? The ones that I find are for chatting and such but I mainly work with tabular EHR data. This is my first foray into this area (LLMs and MoEs) and I am kind of lost with all these Mixtral, openMoE, etc. As we do not have access to Google Collab or have powerful GPUs I have to rely on local training (My lab PC has 2080ti and my laptop has 4070). Any guideline or starting point on how to proceed would be greatly appreciated. submitted by /u/Furiousguy79 [link] [comments]
- [D] What do you use LLMs for?by /u/RND_RandoM (Machine Learning) on July 25, 2024 at 10:31 pm
Just wanted to start a small discussion about why you use LLMs and which model works best for your use case. I am asking because I am concerned that there is little use for LLMs apart from doing role play, helping with coding, and answering general questions submitted by /u/RND_RandoM [link] [comments]
- [R] Moderating LLM Inputs with PromptGuardby /u/Different-General700 (Machine Learning) on July 25, 2024 at 10:12 pm
Meta's release of its latest Llama language model family this week, including the massive Llama-3 405B model, has generated a great deal of excitement among AI developers. These open-weights frontier models, which have been updated with a new license that allows unrestricted use of outputs, will enable significant improvements to AI-powered applications, and enable widespread commercial use of synthetic data. Less discussed, but no less important, are Meta's latest open moderation tools, including a new model called PromptGuard. PromptGuard is a small, lightweight classification model trained to detect malicious prompts, including jailbreaks and prompt injections. These attacks can be used to manipulate language models to produce harmful outputs or extract sensitive information. Companies building enterprise-ready applications must be able to detect and mitigate these attacks to ensure their models are safe to use, especially in sensitive and highly-regulated domains like healthcare, finance, and law. PromptGuard is a text classification model based on mDeBERTa-v3-base, a small transformer model with multilingual capabilities. Meta trained this model to output probabilities for 3 classes: BENIGN, INJECTION, and JAILBREAK. The JAILBREAK class is designed to identify malicious user prompts (such as the "Do Anything Now(opens in a new tab)" or DAN prompt, which instructs a language model to ignore previous instructions and enter an unrestricted mode). On the other hand, the INJECTION class is designed to identify retrieved contexts, such as a webpage or document, which have been poisoned with malicious content to influence the model's output. In our tests, we find that the model is able to identify common jailbreaks like DAN, but also labels benign prompts as injections. This likely happens because the model is trained to handle both prompts and retrieved contexts (such as web searches and news articles), and a benign prompt may appear similar to a malicious context. As stated in the model card: Application developers typically want to allow users flexibility in how they interact with an application, and to only filter explicitly violating prompts (what the ‘jailbreak’ label detects). Third-party content has a different expected distribution of inputs (we don’t expect any “prompt-like” content in this part of the input) This indicates that when applying the model to user prompts, you may want to ignore the INJECTION label, and only filter JAILBREAK inputs. On the other hand, when filtering third-party context to show to the model, such as a news article, you'd want to remove both JAILBREAK and INJECTION labels. We wrote a quick blog post about how you can use PromptGuard to protect your language models from malicious inputs. You can read more here: https://www.trytaylor.ai/blog/promptguard submitted by /u/Different-General700 [link] [comments]
- [P] How to make "Out-of-sample" Predictionsby /u/Individual_Ad_1214 (Machine Learning) on July 25, 2024 at 7:47 pm
My data is a bit complicated to describe so I'm going try to describe something analogous. Each example is randomly generated, but you can group them based on a specific but latent (by latent I mean this isn't added into the features used to develop a model, but I have access to it) feature (in this example we'll call this number of bedrooms). Feature x1 Feature x2 Feature x3 ... Output (Rent) Row 1 Row 2 Row 3 Row 4 Row 5 Row 6 Row 7 2 Row 8 1 Row 9 0 So I can group Row 1, Row 2, and Row 3 based on a latent feature called number of bedrooms (which in this case is 0 bedroom). Similarly, Row 4, Row 5, & Row 6 have 2 Bedrooms, and Row 7, Row 8, & Row 9 have 4 Bedrooms. Furthermore, these groups also have an optimum price which is used to create output classes (output here is Rent; increase, keep constant, or decrease). So say the optimum price for the 4 bedrooms group is $3mil, and row 7 has a price of $4mil (=> 3 - 4 = -1 mil, i.e a -ve value so convert this to class 2, or above optimum or increase rent), row 8 has a price of $3mil (=> 3 - 3 = 0, convert this to class 1, or at optimum), and row 9 has a price of $2mil (3 - 2 = 1, i.e +ve value, so convert this to class 0, or below optimum, or decrease rent). I use this method to create an output class for each example in the dataset (essentially, if example x has y number of bedrooms, I get the known optimum price for that number of bedrooms and I subtract the example's price from the optimum price). Say I have 10 features (e.g. square footage, number of bathrooms, parking spaces etc.) in the dataset, these 10 features provide the model with enough information to figure out the "number of bedrooms". So when I am evaluating the model, feature x1 feature x2 feature x3 ... Row 10 e.g. I pass into the model a test example (Row 10) which I know has 4 bedrooms and is priced at $6mil, the model can accurately predict class 2 (i.e increase rent) for this example. Because the model was developed using data with a representative number of bedrooms in my dataset. Features.... Output (Rent) Row 1 0 Row 2 0 Row 3 0 However, my problem arises at examples with a low number of bedrooms (i.e. 0 bedrooms). The input features doesn't have enough information to determine the number of bedrooms for examples with a low number of bedrooms (which is fine because we assume that within this group, we will always decrease the rent, so we set the optimum price to say $2000. So row 1 price could be $8000, (8000 - 2000 = 6000, +ve value thus convert to class 0 or below optimum/decrease rent). And within this group we rely on the class balance to help the model learn to make predictions because the proportion is heavily skewed towards class 0 (say 95% = class 0 or decrease rent, and 5 % = class 1 or class 2). We do this based the domain knowledge of the data (so in this case, we would always decrease the rent because no one wants to live in a house with 0 bedrooms). MAIN QUESTION: We now want to predict (or undertake inference) for examples with number of bedrooms in between 0 bedrooms and 2 bedrooms (e.g 1 bedroom NOTE: our training data has no example with 1 bedroom). What I notice is that the model's predictions on examples with 1 bedroom act as if these examples had 0 bedrooms and it mostly predicts class 0. My question is, apart from specifically including examples with 1 bedroom in my input data, is there any other way (more statistics or ML related way) for me to improve the ability of my model to generalise on unseen data? submitted by /u/Individual_Ad_1214 [link] [comments]
- [D] Will An Unsupervised FSD Eventually Be Efficient Enough Run on Tesla's HW3?by /u/ZeApelido (Machine Learning) on July 25, 2024 at 7:32 pm
Tesla has a version (V12.5) of their supervised "Full Self Driving" that potential showing signficant improvements, though we will wait to see how much miles per critical disengagment have gone up. (Maybe 600-1000. Previous versions at 100-200 miles per critical disengagement). In order to make this improvement, they upped the parameter count by 5x the previous models. They are just barely making it function on HW3 (works on HW4). These models are already taking advantage of distillation and compression techniques. Considering that the miles per critical disengagement still needs to go up another 100x, I would think model parameter count will have to go up signficantly, maybe 10x-100x? While there are continuing advances in model distillation and compression, I find it hard to fathom that much larger models needed to achieve unsupervised driving will be compressed even further. Tweets like this imply (presumably from advances like LLAMA 2 to LLAMA 3) that these compression ratios will continue at a massive pace. https://x.com/wintonARK/status/1816537413206048915 What do you think? To me, the likely needed increase in model size to get to robotaxi level fidelity will outweigh any advances in distillation so that HW3 will unlikely be able to handle the model. submitted by /u/ZeApelido [link] [comments]
- [R] EMNLP Paper review scoresby /u/Immediate-Hour-8466 (Machine Learning) on July 25, 2024 at 7:06 pm
EMNLP paper review scores Overall assessment for my paper is 2, 2.5 and 3. Is there any chance that it may still be selected? The confidence is 2, 2.5 and 3. The soundness is 2, 2.5, 3.5. I am not sure how soundness and confidence may affect my paper's selection. Pls explain how this works. Which metrics should I consider important. Thank you! submitted by /u/Immediate-Hour-8466 [link] [comments]
- [N] OpenAI announces SearchGPTby /u/we_are_mammals (Machine Learning) on July 25, 2024 at 6:41 pm
https://openai.com/index/searchgpt-prototype/ We’re testing SearchGPT, a temporary prototype of new AI search features that give you fast and timely answers with clear and relevant sources. submitted by /u/we_are_mammals [link] [comments]
- Compute is on Output. Not input. [D]by /u/juliannorton (Machine Learning) on July 25, 2024 at 5:34 pm
Transformer-based LLMs like GPT, Gemini, LLaMa, are decoder-only architectures. This means that the compute utilized and the time it takes for an output is directly related to the size of the output, NOT the size of the input. Meaning you could feed it 100 pages, but if the output is 1 page, that's what matters for compute. It’s notoriously tricky to get an LLM to output text that’s short and concise, and many practitioners give up trying to get it to be concise after some tinkering with prompt engineering. However, fine-tuning deals with the problem at the source, retraining the model to prefer outputting shorter outputs. submitted by /u/juliannorton [link] [comments]
- [P] Local Llama 3.1 and Marqo Retrieval Augmented Generationby /u/elliesleight (Machine Learning) on July 25, 2024 at 4:45 pm
I built a simple starter demo of a Knowledge Question and Answering System using Llama 3.1 (8B GGUF) and Marqo. Feel free to experiment and build on top of this yourselves! GitHub: https://github.com/ellie-sleightholm/marqo-llama3_1 submitted by /u/elliesleight [link] [comments]
- [N] AI achieves silver-medal standard solving International Mathematical Olympiad problemsby /u/we_are_mammals (Machine Learning) on July 25, 2024 at 4:16 pm
https://deepmind.google/discover/blog/ai-solves-imo-problems-at-silver-medal-level/ They solved 4 of the 6 IMO problems (although it took days to solve some of them). This would have gotten them a score of 28/42, just one point below the gold-medal level. submitted by /u/we_are_mammals [link] [comments]
- [R] Explainability of HuggingFace Models (LLMs) for Text Summarization/Generation Tasksby /u/PhoenixHeadshot25 (Machine Learning) on July 25, 2024 at 3:19 pm
Hi community, I am exploring the Responsible AI domain where I have started reading about methods and tools to make Deep Learning Models explainable. I have already used SHAP and LIMe for ML model explainability. However, I am unsure about their use in explaining LLMs. I know that these methods are model agnostic but can we use these methods for Text Generation or Summarization tasks? I got reference docs from Shap explaining GPT2 for text generation tasks, but I am unsure about using it for other newer LLMs. Additionally, I would like to know, are there any better ways for Explainable AI for LLMs? submitted by /u/PhoenixHeadshot25 [link] [comments]
- [D] High-Dimensional Probabilistic Modelsby /u/smorad (Machine Learning) on July 25, 2024 at 2:58 pm
What is the standard way to model high-dimensional stochastic processes today? I have some process defined over images x, and I would like to compute P(x' | x, z) for all x'. I know there are Normalizing Flows, Gaussian Processes, etc, but I do not know which to get started with. I specifically want to compute the probabilities, not just sample some x' ~ P(x, z). submitted by /u/smorad [link] [comments]
- [R] Shared Imagination: LLMs Hallucinate Alikeby /u/zyl1024 (Machine Learning) on July 25, 2024 at 1:49 pm
Happy to share our recent paper, where we demonstrate that LLMs exhibit surprising agreement on purely imaginary and hallucinated contents -- what we call a "shared imagination space". To arrive at this conclusion, we ask LLMs to generate questions on hypothetical contents (e.g., a made-up concept in physics) and then find that they can answer each other's (unanswerable and nonsensical) questions with much higher accuracy than random chance. From this, we investigate in multiple directions on its emergence, generality and possible reasons, and given such consistent hallucination and imagination behavior across modern LLMs, discuss implications to hallucination detection and computational creativity. Link to the paper: https://arxiv.org/abs/2407.16604 Link to the tweet with result summary and highlight: https://x.com/YilunZhou/status/1816371178501476473 Please feel free to ask any questions! The main experiment setup and finding. submitted by /u/zyl1024 [link] [comments]
- [R] Paper NAACL 2024: "Reliability Estimation of News Media Sources: Birds of a Feather Flock Together"by /u/sergbur (Machine Learning) on July 25, 2024 at 9:10 am
For people working on information verification in general, for instance, working on fact checking, fake news detection or even using RAG from news articles this paper may be useful. Authors use different reinforcement learning techniques to estimate reliability values of news media outlets based on how they interact on the web. The method is easy to scale since the source code is available to build larger hyperlink-based interaction graphs from Common Crawl News. Authors also released the computed values and dataset with news media reliability annotation: Github repo: https://github.com/idiap/News-Media-Reliability Paper: https://aclanthology.org/2024.naacl-long.383/ Live Demo Example: https://lab.idiap.ch/criteria/ In the demo, the retrieved news articles will be order not only by the match to the query but also by the estimated reliability for each sources (URL domains are color coded from green to red, for instance, scrolling down will show results coming from less reliable sources marked with red-ish colors). Alternatively, if a news URL or a news outlet domain (e.g. apnews.com) is given as a query, information about the estimated values are detailed (e.g. showing the neighboring sources interacting with the media, etc.) Have a nice day, everyone! 🙂 submitted by /u/sergbur [link] [comments]
- [D] ACL ARR June (EMNLP) Review Discussionby /u/always_been_a_toy (Machine Learning) on July 25, 2024 at 4:45 am
Too anxious about reviews as they didn’t arrive yet! Wanted to share with the community and see the reactions to the reviews! Rant and stuff! Be polite in comments. submitted by /u/always_been_a_toy [link] [comments]
- "[Discussion]" Where do you get your updates on latest research in video generation and computer vision?by /u/Sobieski526 (Machine Learning) on July 24, 2024 at 9:20 pm
As the title says, looking for some tips on how you keep track of the latest research in video generation and CV. I have been reading through https://cvpr.thecvf.com/ and it's a great source, are there any simiar ones? submitted by /u/Sobieski526 [link] [comments]
- [R] Pre-prompting your LLM increases performanceby /u/CalendarVarious3992 (Machine Learning) on July 24, 2024 at 8:33 pm
Research done at UoW shows that pre-prompting your LLM, or providing context prior to asking your question leads to better results. Even when the context is self generated. https://arxiv.org/pdf/2110.08387 For example asking, "What should I do while in Rome?" is less effective than a series of prompts, "What are the top restaraunts in Rome?" "What are the top sight seeing locations in Rome?" "Best things to do in Rome" "What should I do in Rome?" I always figured this was the case from anecdotal evidence but good to see people who are way starter than me explain it in this paper. And while chain prompting is a little more time consuming there's chrome extensions like ChatGPT Queue that ease up the process. Are their any other "hacks" to squeeze out better performance ? submitted by /u/CalendarVarious3992 [link] [comments]
- [R] Segment Anything Repository Archived - Why?by /u/Ben-L-921 (Machine Learning) on July 24, 2024 at 8:23 pm
Hello ML subreddit, I was recently made aware of the fact that the segment anything repository got made into a public archive less than a month ago (July 1st, 2024). I was not able to find any information pertaining to why this was the case, however. I know there have been a lot of derivatives of segment anything in development, but I don't know why this would have warranted a public archive. Does anyone know why this happened and where we might be able to redirect questions/issues for the work? submitted by /u/Ben-L-921 [link] [comments]
- [N] Mistral releases a "Large Enough" modelby /u/we_are_mammals (Machine Learning) on July 24, 2024 at 7:04 pm
https://mistral.ai/news/mistral-large-2407/ 123B parameters On par with GPT-4o and Llama 3.1 405B, according to their benchmarks Mistral Research License allows usage and modification for research and non-commercial purposes submitted by /u/we_are_mammals [link] [comments]
- [P] NCCLX mentioned in llama3 paperby /u/khidot (Machine Learning) on July 24, 2024 at 3:54 pm
The paper says `Our collective communication library for Llama 3 is based on a fork of Nvidia’s NCCL library, called NCCLX. NCCLX significantly improves the performance of NCCL, especially for higher latency networks`. Can anyone give more background? Any plans to release or upstream? Any more technical details? submitted by /u/khidot [link] [comments]
Active Hydrating Toner, Anti-Aging Replenishing Advanced Face Moisturizer, with Vitamins A, C, E & Natural Botanicals to Promote Skin Balance & Collagen Production, 6.7 Fl Oz
Age Defying 0.3% Retinol Serum, Anti-Aging Dark Spot Remover for Face, Fine Lines & Wrinkle Pore Minimizer, with Vitamin E & Natural Botanicals
Firming Moisturizer, Advanced Hydrating Facial Replenishing Cream, with Hyaluronic Acid, Resveratrol & Natural Botanicals to Restore Skin's Strength, Radiance, and Resilience, 1.75 Oz
Skin Stem Cell Serum
Smartphone 101 - Pick a smartphone for me - android or iOS - Apple iPhone or Samsung Galaxy or Huawei or Xaomi or Google Pixel
Can AI Really Predict Lottery Results? We Asked an Expert.
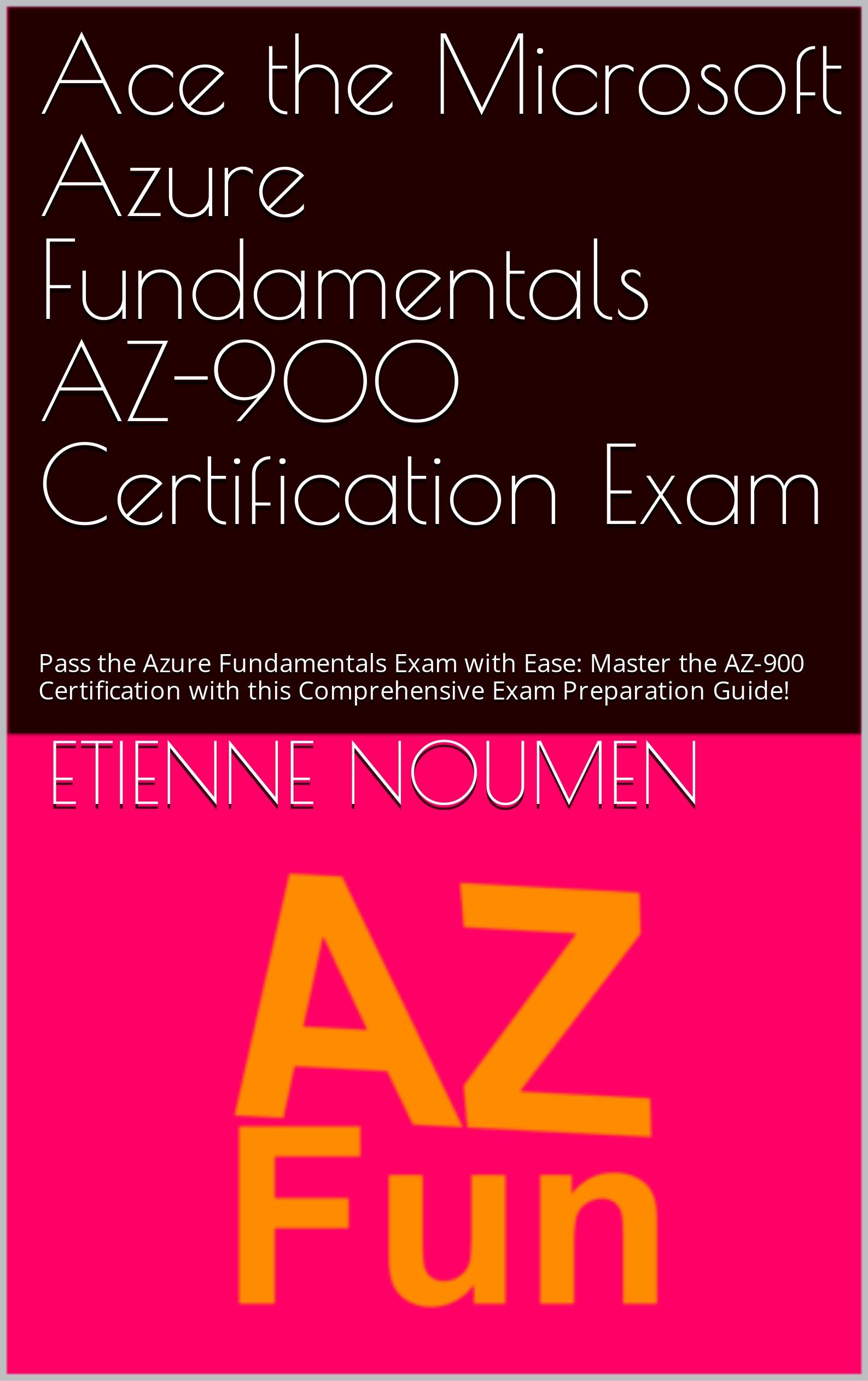

Djamgatech

Read Photos and PDFs Aloud for me iOS
Read Photos and PDFs Aloud for me android
Read Photos and PDFs Aloud For me Windows 10/11
Read Photos and PDFs Aloud For Amazon
Get 20% off Google Workspace (Google Meet) Business Plan (AMERICAS): M9HNXHX3WC9H7YE (Email us for more)
Get 20% off Google Google Workspace (Google Meet) Standard Plan with the following codes: 96DRHDRA9J7GTN6(Email us for more)
FREE 10000+ Quiz Trivia and and Brain Teasers for All Topics including Cloud Computing, General Knowledge, History, Television, Music, Art, Science, Movies, Films, US History, Soccer Football, World Cup, Data Science, Machine Learning, Geography, etc....

List of Freely available programming books - What is the single most influential book every Programmers should read
- Bjarne Stroustrup - The C++ Programming Language
- Brian W. Kernighan, Rob Pike - The Practice of Programming
- Donald Knuth - The Art of Computer Programming
- Ellen Ullman - Close to the Machine
- Ellis Horowitz - Fundamentals of Computer Algorithms
- Eric Raymond - The Art of Unix Programming
- Gerald M. Weinberg - The Psychology of Computer Programming
- James Gosling - The Java Programming Language
- Joel Spolsky - The Best Software Writing I
- Keith Curtis - After the Software Wars
- Richard M. Stallman - Free Software, Free Society
- Richard P. Gabriel - Patterns of Software
- Richard P. Gabriel - Innovation Happens Elsewhere
- Code Complete (2nd edition) by Steve McConnell
- The Pragmatic Programmer
- Structure and Interpretation of Computer Programs
- The C Programming Language by Kernighan and Ritchie
- Introduction to Algorithms by Cormen, Leiserson, Rivest & Stein
- Design Patterns by the Gang of Four
- Refactoring: Improving the Design of Existing Code
- The Mythical Man Month
- The Art of Computer Programming by Donald Knuth
- Compilers: Principles, Techniques and Tools by Alfred V. Aho, Ravi Sethi and Jeffrey D. Ullman
- Gödel, Escher, Bach by Douglas Hofstadter
- Clean Code: A Handbook of Agile Software Craftsmanship by Robert C. Martin
- Effective C++
- More Effective C++
- CODE by Charles Petzold
- Programming Pearls by Jon Bentley
- Working Effectively with Legacy Code by Michael C. Feathers
- Peopleware by Demarco and Lister
- Coders at Work by Peter Seibel
- Surely You're Joking, Mr. Feynman!
- Effective Java 2nd edition
- Patterns of Enterprise Application Architecture by Martin Fowler
- The Little Schemer
- The Seasoned Schemer
- Why's (Poignant) Guide to Ruby
- The Inmates Are Running The Asylum: Why High Tech Products Drive Us Crazy and How to Restore the Sanity
- The Art of Unix Programming
- Test-Driven Development: By Example by Kent Beck
- Practices of an Agile Developer
- Don't Make Me Think
- Agile Software Development, Principles, Patterns, and Practices by Robert C. Martin
- Domain Driven Designs by Eric Evans
- The Design of Everyday Things by Donald Norman
- Modern C++ Design by Andrei Alexandrescu
- Best Software Writing I by Joel Spolsky
- The Practice of Programming by Kernighan and Pike
- Pragmatic Thinking and Learning: Refactor Your Wetware by Andy Hunt
- Software Estimation: Demystifying the Black Art by Steve McConnel
- The Passionate Programmer (My Job Went To India) by Chad Fowler
- Hackers: Heroes of the Computer Revolution
- Algorithms + Data Structures = Programs
- Writing Solid Code
- JavaScript - The Good Parts
- Getting Real by 37 Signals
- Foundations of Programming by Karl Seguin
- Computer Graphics: Principles and Practice in C (2nd Edition)
- Thinking in Java by Bruce Eckel
- The Elements of Computing Systems
- Refactoring to Patterns by Joshua Kerievsky
- Modern Operating Systems by Andrew S. Tanenbaum
- The Annotated Turing
- Things That Make Us Smart by Donald Norman
- The Timeless Way of Building by Christopher Alexander
- The Deadline: A Novel About Project Management by Tom DeMarco
- The C++ Programming Language (3rd edition) by Stroustrup
- Patterns of Enterprise Application Architecture
- Computer Systems - A Programmer's Perspective
- Agile Principles, Patterns, and Practices in C# by Robert C. Martin
- Growing Object-Oriented Software, Guided by Tests
- Framework Design Guidelines by Brad Abrams
- Object Thinking by Dr. David West
- Advanced Programming in the UNIX Environment by W. Richard Stevens
- Hackers and Painters: Big Ideas from the Computer Age
- The Soul of a New Machine by Tracy Kidder
- CLR via C# by Jeffrey Richter
- The Timeless Way of Building by Christopher Alexander
- Design Patterns in C# by Steve Metsker
- Alice in Wonderland by Lewis Carol
- Zen and the Art of Motorcycle Maintenance by Robert M. Pirsig
- About Face - The Essentials of Interaction Design
- Here Comes Everybody: The Power of Organizing Without Organizations by Clay Shirky
- The Tao of Programming
- Computational Beauty of Nature
- Writing Solid Code by Steve Maguire
- Philip and Alex's Guide to Web Publishing
- Object-Oriented Analysis and Design with Applications by Grady Booch
- Effective Java by Joshua Bloch
- Computability by N. J. Cutland
- Masterminds of Programming
- The Tao Te Ching
- The Productive Programmer
- The Art of Deception by Kevin Mitnick
- The Career Programmer: Guerilla Tactics for an Imperfect World by Christopher Duncan
- Paradigms of Artificial Intelligence Programming: Case studies in Common Lisp
- Masters of Doom
- Pragmatic Unit Testing in C# with NUnit by Andy Hunt and Dave Thomas with Matt Hargett
- How To Solve It by George Polya
- The Alchemist by Paulo Coelho
- Smalltalk-80: The Language and its Implementation
- Writing Secure Code (2nd Edition) by Michael Howard
- Introduction to Functional Programming by Philip Wadler and Richard Bird
- No Bugs! by David Thielen
- Rework by Jason Freid and DHH
- JUnit in Action
#BlackOwned #BlackEntrepreneurs #BlackBuniness #AWSCertified #AWSCloudPractitioner #AWSCertification #AWSCLFC02 #CloudComputing #AWSStudyGuide #AWSTraining #AWSCareer #AWSExamPrep #AWSCommunity #AWSEducation #AWSBasics #AWSCertified #AWSMachineLearning #AWSCertification #AWSSpecialty #MachineLearning #AWSStudyGuide #CloudComputing #DataScience #AWSCertified #AWSSolutionsArchitect #AWSArchitectAssociate #AWSCertification #AWSStudyGuide #CloudComputing #AWSArchitecture #AWSTraining #AWSCareer #AWSExamPrep #AWSCommunity #AWSEducation #AzureFundamentals #AZ900 #MicrosoftAzure #ITCertification #CertificationPrep #StudyMaterials #TechLearning #MicrosoftCertified #AzureCertification #TechBooks
Top 1000 Canada Quiz and trivia: CANADA CITIZENSHIP TEST- HISTORY - GEOGRAPHY - GOVERNMENT- CULTURE - PEOPLE - LANGUAGES - TRAVEL - WILDLIFE - HOCKEY - TOURISM - SCENERIES - ARTS - DATA VISUALIZATION

Top 1000 Africa Quiz and trivia: HISTORY - GEOGRAPHY - WILDLIFE - CULTURE - PEOPLE - LANGUAGES - TRAVEL - TOURISM - SCENERIES - ARTS - DATA VISUALIZATION

Exploring the Pros and Cons of Visiting All Provinces and Territories in Canada.

Exploring the Advantages and Disadvantages of Visiting All 50 States in the USA

Health Health, a science-based community to discuss health news and the coronavirus (COVID-19) pandemic
- US infant mortality increased in 2022 for the first time in decades, CDC report showsby /u/cnn on July 25, 2024 at 6:37 pm
submitted by /u/cnn [link] [comments]
- Study raises hopes that shingles vaccine may delay onset of dementia | Dementia | The Guardianby /u/chilladipa on July 25, 2024 at 3:38 pm
submitted by /u/chilladipa [link] [comments]
- How fit is your city? New rankings by the American College of Sports Medicineby /u/idc2011 on July 25, 2024 at 3:35 pm
submitted by /u/idc2011 [link] [comments]
- Twice-Yearly Lenacapavir or Daily F/TAF for HIV Prevention in Cisgender Women | New England Journal of Medicineby /u/chilladipa on July 25, 2024 at 3:30 pm
submitted by /u/chilladipa [link] [comments]
- Biden Made a Healthy Decisionby /u/theatlantic on July 25, 2024 at 3:15 pm
submitted by /u/theatlantic [link] [comments]
Today I Learned (TIL) You learn something new every day; what did you learn today? Submit interesting and specific facts about something that you just found out here.
- TIL actor John Larroquette was the uncredited narrator of the prologue to the 1974 horror movie Texas Chainsaw Massacre. In lieu of cash, he was paid by the Director Tobe Hooper in Marijuana.by /u/openletter8 on July 25, 2024 at 6:56 pm
submitted by /u/openletter8 [link] [comments]
- TIL that the every Shakopee Mdewakanton Sioux indian receives a payout of around $1 million per year from casino profits.by /u/friendlystranger4u on July 25, 2024 at 6:22 pm
submitted by /u/friendlystranger4u [link] [comments]
- TIL Motorcycles in China are dictated by law to be decommissioned and destroyed in 13 years after registration regardless of the conditionsby /u/Easy_Piece_592 on July 25, 2024 at 5:56 pm
submitted by /u/Easy_Piece_592 [link] [comments]
- TIL a man named Jonathan Riches has filed more than 2,600 lawsuits since 2006. He even sued Guinness World Records to try to stop them from titling him as "the most litigious man in history".by /u/doopityWoop22 on July 25, 2024 at 5:03 pm
submitted by /u/doopityWoop22 [link] [comments]
- TIL that in 2018, an American half-pipe skier qualified for the Olympics despite minimal experience. Olympic requirements stated that an athlete needed to place in the top 30 at multiple events. She simply sought out events with fewer than 30 participants, showed up, and skied down without falling.by /u/ctdca on July 25, 2024 at 4:28 pm
submitted by /u/ctdca [link] [comments]
Reddit Science This community is a place to share and discuss new scientific research. Read about the latest advances in astronomy, biology, medicine, physics, social science, and more. Find and submit new publications and popular science coverage of current research.
- Abstinence-only sex education linked to higher pornography use among women | This finding adds to the ongoing conversation about the effectiveness and impacts of different sexuality education approaches.by /u/chrisdh79 on July 25, 2024 at 6:49 pm
submitted by /u/chrisdh79 [link] [comments]
- AlphaProof and AlphaGeometry 2 AI models achieve silver medal standard in solving International Mathematical Olympiad problemsby /u/Big_Profit9076 on July 25, 2024 at 5:59 pm
submitted by /u/Big_Profit9076 [link] [comments]
- Scientists have described a new species of chordate, Nuucichthys rhynchocephalus, the first soft-bodied vertebrate from the Drumian Marjum Formation of the American Great Basin.by /u/grimisgreedy on July 25, 2024 at 5:55 pm
submitted by /u/grimisgreedy [link] [comments]
- Secularists revealed as a unique political force in America, with an intriguing divergence from liberals. Unlike nonreligiosity, which denotes a lack of religious affiliation or belief, secularism involves an active identification with principles grounded in empirical evidence and rational thought.by /u/mvea on July 25, 2024 at 5:40 pm
submitted by /u/mvea [link] [comments]
- New shingles vaccine could reduce risk of dementia. The study found at least a 17% reduction in dementia diagnoses in the six years after the new recombinant shingles vaccination, equating to 164 or more additional days lived without dementia.by /u/Wagamaga on July 25, 2024 at 4:48 pm
submitted by /u/Wagamaga [link] [comments]
Reddit Sports Sports News and Highlights from the NFL, NBA, NHL, MLB, MLS, and leagues around the world.
- A's place their lone all-star, Mason Miller, on IL with fractured finger after hitting training tableby /u/Oldtimer_2 on July 25, 2024 at 8:15 pm
submitted by /u/Oldtimer_2 [link] [comments]
- Flyers sign All-Star Travis Konecny to an 8-year extension worth $70 millionby /u/Oldtimer_2 on July 25, 2024 at 7:45 pm
submitted by /u/Oldtimer_2 [link] [comments]
- Bills’ Von Miller says he believes domestic assault case to be closed, with no charges filedby /u/Oldtimer_2 on July 25, 2024 at 7:43 pm
submitted by /u/Oldtimer_2 [link] [comments]
- Padres' Dylan Cease throws no-hitter vs. Nationalsby /u/Oldtimer_2 on July 25, 2024 at 7:41 pm
submitted by /u/Oldtimer_2 [link] [comments]
- Appeal denied in Valieva case; U.S. skaters to get gold in Parisby /u/PrincessBananas85 on July 25, 2024 at 6:18 pm
submitted by /u/PrincessBananas85 [link] [comments]