AI Dashboard is available on the Web, Apple, Google, and Microsoft, PRO version
Join us as we explore the fascinating intertwine of Generative and Discriminative AI, two groundbreaking domains in the tech realm. Signal’s CEO, David Benigson, shares expert insights, discussing the imperative balance and collaboration between these AI subsets. Don’t miss out on an enlightening conversation with one of the industry’s brightest minds!

Welcome to AI Unraveled, the podcast that demystifies frequently asked questions on artificial intelligence and keeps you up to date with the latest AI trends. Join us as we delve into groundbreaking research, innovative applications, and emerging technologies that are pushing the boundaries of AI. From the latest trends in ChatGPT and the recent merger of Google Brain and DeepMind, to the exciting developments in generative AI, we’ve got you covered with a comprehensive update on the ever-evolving AI landscape. In today’s episode, we’ll cover the different applications of generative and discriminative AI, Signal AI’s role in pioneering discriminative AI, how discriminative AI improves Signal AI’s evaluation, addressing reliability concerns of generative AI, how generative and discriminative AI combine to expand business opportunities, the effectiveness of generative and discriminative AI for decision-making, the challenges Signal AI faces in integrating and optimizing AI, choosing an AI partner wisely, and exciting features of the podcast by Wondercraft AI and the book “AI Unraveled” by Etienne Noumen.

Table of Contents
Get 20% off Google Google Workspace (Google Meet) Standard Plan with the following codes: 96DRHDRA9J7GTN6
Get 20% off Google Workspace (Google Meet) Business Plan (AMERICAS): M9HNXHX3WC9H7YE (Email us for more codes)
Active Anti-Aging Eye Gel, Reduces Dark Circles, Puffy Eyes, Crow's Feet and Fine Lines & Wrinkles, Packed with Hyaluronic Acid & Age Defying Botanicals
Defining the Landscape: David, can you start by clarifying for our listeners the core differences between generative AI and discriminative AI and why understanding these differences is crucial for businesses today?
Discriminative AI learns to distinguish between different classes of data Generative AI learns to create new data similar to the data it was trained on.
For business, both generative and discriminative AI are both powerful tools that can be used to solve a wide variety of problems – which type of AI you use and how you use it will depend on the specific task that you are trying to accomplish.
Generative AI models are typically better at tasks that require creativity or imagination. For example, generative AI models can be used to generate new images, text, or music. Alternatively, discriminative AI models are typically better at tasks that require accurate classification or prediction.
Historical Perspective: Signal AI has been in the AI space for a decade. How have you seen the landscape evolve, specifically with regard to discriminative AI?
Over a decade ago, Signal AI was founded in an auto repair shop with a mission to create a better way for brands to track their media mentions. Departing from the limitations of Boolean queries, Signal AI innovated an industry-first media monitoring platform entirely based on AI-trained discriminative models that automatically identify media hits mentioning an organization, topic, person, or a range of other entities. We’ve achieved an incredible degree of fidelity with our model trained on more than four billion documents. This capability also powers our sentiment analysis engine, which is the only one on the market capable of identifying the subject (entity) of a statement. Just this year, Signal AI customers have used more than 88,000 AI-trained entities, saving more than 400,000 hours of work.
We remain the only true AI-powered challenger in a field of legacy dinosaurs. With over ten years’ worth of AI-trained topics and entities under our belt, we can derive rich insights not only from the media we’re tracking, but also from the relational metadata between stories. This relational analysis is unmatched in our industry, going far beyond the mere tallying of media hits. This relational analysis is unmatched in the industry – it enables us to generate powerful signals, such as identifying a company’s affinity on a topic, understanding which events have the greatest impact on a brand, and uncovering highly-granular messaging whitespace.
Evaluative Capabilities: How does discriminative AI enhance Signal AI’s ability to evaluate and categorize new, often voluminous, information efficiently?
Discriminative AI enhances Signal AI’s efficiency in evaluating and categorizing vast amounts of new data. Unlike generative AI, which generates content, discriminative AI specializes in evaluating and distinguishing information, such as differentiating between contexts like the fruit “apple” and the tech company “Apple”. In a world flooded with AI-generated content, discriminative AI augments generative AI by expanding its use cases, aiding decision-makers in pinpointing brand strategies for maximum impact. When combined with generative AI, discriminative AI accelerates strategic decision-making by evaluating and categorizing data swiftly and accurately. While generative AI might introduce risks like misinformation, pairing it with discriminative AI enhances trustworthiness.
Trust in Generative AI: With the advancements in generative AI, there’s a growing concern about its reliability. How does pairing it with discriminative AI address these concerns and ensure that the information is both innovative and factual?
Generative AI has seen rapid advancements even in just the last several months, but its reliability remains a concern. Pairing it with discriminative AI, which excels in evaluating and categorizing content, can address many of these reliability concerns. While generative AI excels at generating content, discriminative AI ensures it’s grounded in facts, acting as a check for accuracy. This pairing also offers broader applications – for example, generative AI can help synthesize insights from large data sets, while discriminative AI validates them. Risks inherent to generative AI, like hallucinations and misinformation, are mitigated when coupled with the evaluative strength of discriminative AI.
Broadening Horizons: Can you share a use case where the combination of generative and discriminative AI significantly expanded the potential applications or outcomes for a client?
Combining generative and discriminative AI opens up tremendous opportunities for businesses.
One use case highlighting this synergy involves strategic brand positioning for companies. Discriminative AI, with its prowess in evaluating and categorizing content, is great at mapping new narrative whitespaces and enabling brands to find the right message to connect authentically with audiences. Meanwhile, generative AI, with its ability to interpret inputs and generate text, can help customers evaluate the meaning behind charts and datasets, quickly pulling out key actionable insights.
Together, these AI forms not only enhance data-driven decision-making but also offer an expanded horizon of possibilities. For a client, this combination means not just having creative strategies but ensuring those strategies are grounded in factual, data-driven insights, leading to more fruitful outcomes.
Hallucinations in AI: There’s a lot of talk about AI “hallucinations” or generating misleading outputs. How does discriminative AI act as a check on this, ensuring that the generated content aligns with reality?
We’ve increasingly seen conversations about how generative AI can sometimes produce “hallucinations” or misleading outputs. Discriminative AI, on the other hand, specializes in evaluating and categorizing content, discerning what aligns with facts and reality. By pairing generative AI with discriminative AI, the latter has the potential to act as a check, which will ensure that the generated content is accurate.
Optimizing Collaboration: If we were to personify generative AI as the “creative friend” and discriminative AI as the “fact-focused buddy”, how do you ensure they ‘work together’ effectively at Signal AI?
Please see other answers that speak to this topic specifically.
Future Vision: Looking forward, how do you envision the relationship between generative and discriminative AI evolving, especially in the context of business decision-making?
As businesses increasingly lean on AI for decision-making, the relationship between generative and discriminative AI will only become more intertwined. Generative AI, known for its creativity, will continue to craft innovative insights while discriminative AI will play an important role in evaluating and validating the generated content.
This synergistic relationship will lead to smarter strategies. For example, in brand positioning, generative AI might suggest novel narratives, with discriminative AI ensuring that any findings are grounded in validated data and facts.
Challenges Ahead: What are some of the challenges Signal AI faces in further integrating and optimizing these two forms of AI, and how are you working to overcome them?
As we continue to see with tools like ChatGPT, one of the biggest challenges facing the AI industry as a whole is data accuracy and reliability. While discriminative AI can play a key role in validating datasets, AI vendors must also do a better job of licensing high-quality content and fairly compensating content creators. Both generative and discriminative AI depend on a thriving content ecosystem to function – safeguarding this should be top of mind for every AI firm.
Words of Wisdom: Lastly, for businesses just starting to explore the potential of AI, especially discriminative AI, what advice would you give them as they embark on this journey?
Few technology decisions will carry more gravity for businesses in the next 10 years than their choice of AI partner. Avoid cynical vendors using AI as a shiny wrapper to repackage the same old legacy software. Don’t be hoodwinked. Ask: does this company truly have a legacy in AI innovation and research?
Unraveling the Symbiosis of Generative and Discriminative AI: Conclusion
In this episode, we explored how generative and discriminative AI work together to create accurate and innovative data, how Signal AI has been pioneering discriminative AI for a decade, and the challenges of integrating and optimizing these technologies. We also learned the importance of choosing an AI partner wisely.
This podcast is generated using the Wondercraft AI platform (https://www.wondercraft.ai/?via=etienne), a tool that makes it super easy to start your own podcast, by enabling you to use hyper-realistic AI voices as your host. Like mine!
Attention AI Unraveled podcast listeners!
Are you eager to expand your understanding of artificial intelligence? Look no further than the essential book “AI Unraveled: Demystifying Frequently Asked Questions on Artificial Intelligence,” by Etienne Noumen, now available at Shopify, Apple, Google, or Amazon (https://amzn.to/44Y5u3y) today!
OpenAI’s ChatGPT enters classrooms and could AI be the game-changer in tackling the opioid epidemic?
Active Hydrating Toner, Anti-Aging Replenishing Advanced Face Moisturizer, with Vitamins A, C, E & Natural Botanicals to Promote Skin Balance & Collagen Production, 6.7 Fl Oz
Age Defying 0.3% Retinol Serum, Anti-Aging Dark Spot Remover for Face, Fine Lines & Wrinkle Pore Minimizer, with Vitamin E & Natural Botanicals
Firming Moisturizer, Advanced Hydrating Facial Replenishing Cream, with Hyaluronic Acid, Resveratrol & Natural Botanicals to Restore Skin's Strength, Radiance, and Resilience, 1.75 Oz
Skin Stem Cell Serum
Smartphone 101 - Pick a smartphone for me - android or iOS - Apple iPhone or Samsung Galaxy or Huawei or Xaomi or Google Pixel
Can AI Really Predict Lottery Results? We Asked an Expert.
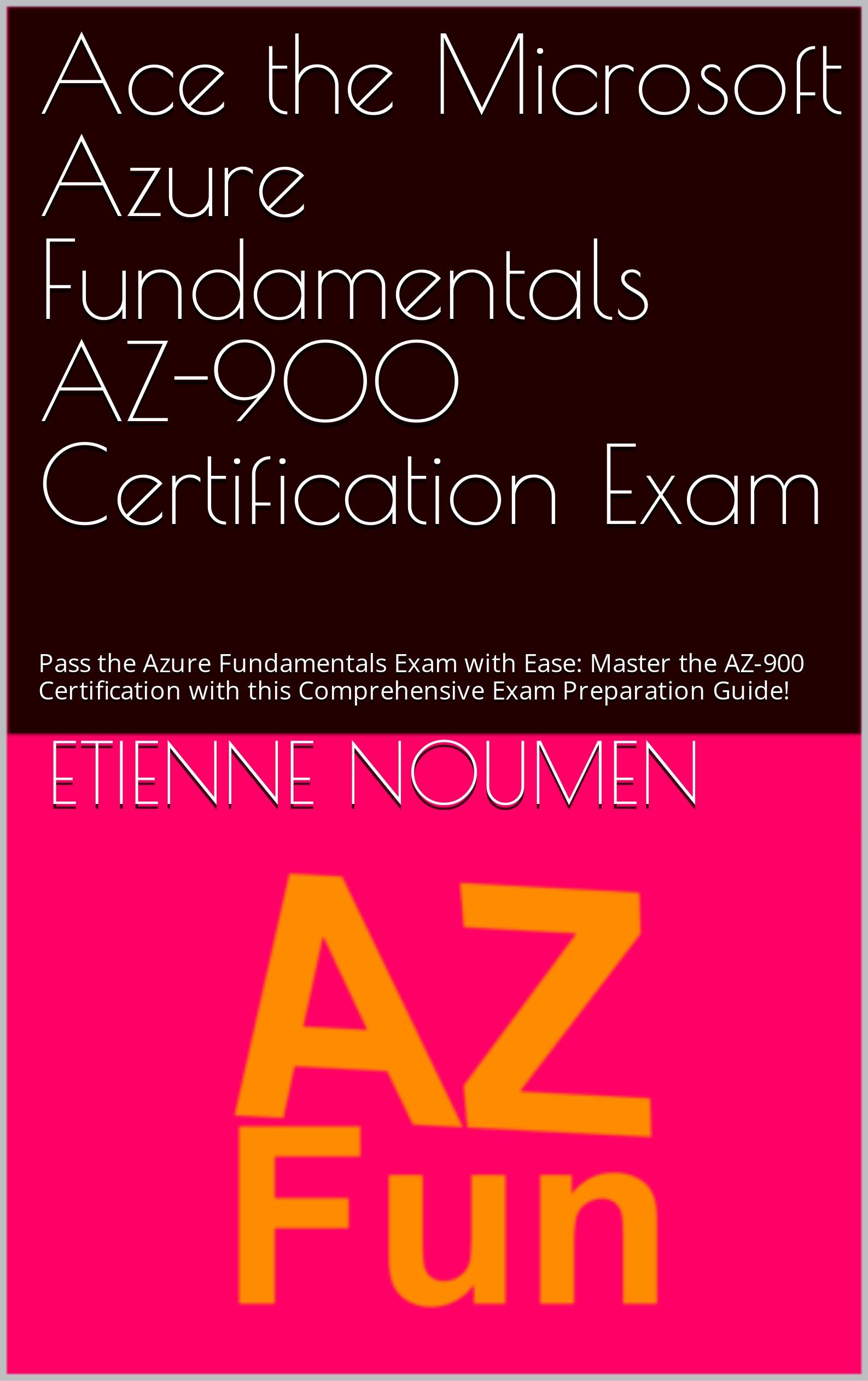

Djamgatech

Read Photos and PDFs Aloud for me iOS
Read Photos and PDFs Aloud for me android
Read Photos and PDFs Aloud For me Windows 10/11
Read Photos and PDFs Aloud For Amazon
Get 20% off Google Workspace (Google Meet) Business Plan (AMERICAS): M9HNXHX3WC9H7YE (Email us for more)
Get 20% off Google Google Workspace (Google Meet) Standard Plan with the following codes: 96DRHDRA9J7GTN6(Email us for more)
FREE 10000+ Quiz Trivia and and Brain Teasers for All Topics including Cloud Computing, General Knowledge, History, Television, Music, Art, Science, Movies, Films, US History, Soccer Football, World Cup, Data Science, Machine Learning, Geography, etc....

List of Freely available programming books - What is the single most influential book every Programmers should read
- Bjarne Stroustrup - The C++ Programming Language
- Brian W. Kernighan, Rob Pike - The Practice of Programming
- Donald Knuth - The Art of Computer Programming
- Ellen Ullman - Close to the Machine
- Ellis Horowitz - Fundamentals of Computer Algorithms
- Eric Raymond - The Art of Unix Programming
- Gerald M. Weinberg - The Psychology of Computer Programming
- James Gosling - The Java Programming Language
- Joel Spolsky - The Best Software Writing I
- Keith Curtis - After the Software Wars
- Richard M. Stallman - Free Software, Free Society
- Richard P. Gabriel - Patterns of Software
- Richard P. Gabriel - Innovation Happens Elsewhere
- Code Complete (2nd edition) by Steve McConnell
- The Pragmatic Programmer
- Structure and Interpretation of Computer Programs
- The C Programming Language by Kernighan and Ritchie
- Introduction to Algorithms by Cormen, Leiserson, Rivest & Stein
- Design Patterns by the Gang of Four
- Refactoring: Improving the Design of Existing Code
- The Mythical Man Month
- The Art of Computer Programming by Donald Knuth
- Compilers: Principles, Techniques and Tools by Alfred V. Aho, Ravi Sethi and Jeffrey D. Ullman
- Gödel, Escher, Bach by Douglas Hofstadter
- Clean Code: A Handbook of Agile Software Craftsmanship by Robert C. Martin
- Effective C++
- More Effective C++
- CODE by Charles Petzold
- Programming Pearls by Jon Bentley
- Working Effectively with Legacy Code by Michael C. Feathers
- Peopleware by Demarco and Lister
- Coders at Work by Peter Seibel
- Surely You're Joking, Mr. Feynman!
- Effective Java 2nd edition
- Patterns of Enterprise Application Architecture by Martin Fowler
- The Little Schemer
- The Seasoned Schemer
- Why's (Poignant) Guide to Ruby
- The Inmates Are Running The Asylum: Why High Tech Products Drive Us Crazy and How to Restore the Sanity
- The Art of Unix Programming
- Test-Driven Development: By Example by Kent Beck
- Practices of an Agile Developer
- Don't Make Me Think
- Agile Software Development, Principles, Patterns, and Practices by Robert C. Martin
- Domain Driven Designs by Eric Evans
- The Design of Everyday Things by Donald Norman
- Modern C++ Design by Andrei Alexandrescu
- Best Software Writing I by Joel Spolsky
- The Practice of Programming by Kernighan and Pike
- Pragmatic Thinking and Learning: Refactor Your Wetware by Andy Hunt
- Software Estimation: Demystifying the Black Art by Steve McConnel
- The Passionate Programmer (My Job Went To India) by Chad Fowler
- Hackers: Heroes of the Computer Revolution
- Algorithms + Data Structures = Programs
- Writing Solid Code
- JavaScript - The Good Parts
- Getting Real by 37 Signals
- Foundations of Programming by Karl Seguin
- Computer Graphics: Principles and Practice in C (2nd Edition)
- Thinking in Java by Bruce Eckel
- The Elements of Computing Systems
- Refactoring to Patterns by Joshua Kerievsky
- Modern Operating Systems by Andrew S. Tanenbaum
- The Annotated Turing
- Things That Make Us Smart by Donald Norman
- The Timeless Way of Building by Christopher Alexander
- The Deadline: A Novel About Project Management by Tom DeMarco
- The C++ Programming Language (3rd edition) by Stroustrup
- Patterns of Enterprise Application Architecture
- Computer Systems - A Programmer's Perspective
- Agile Principles, Patterns, and Practices in C# by Robert C. Martin
- Growing Object-Oriented Software, Guided by Tests
- Framework Design Guidelines by Brad Abrams
- Object Thinking by Dr. David West
- Advanced Programming in the UNIX Environment by W. Richard Stevens
- Hackers and Painters: Big Ideas from the Computer Age
- The Soul of a New Machine by Tracy Kidder
- CLR via C# by Jeffrey Richter
- The Timeless Way of Building by Christopher Alexander
- Design Patterns in C# by Steve Metsker
- Alice in Wonderland by Lewis Carol
- Zen and the Art of Motorcycle Maintenance by Robert M. Pirsig
- About Face - The Essentials of Interaction Design
- Here Comes Everybody: The Power of Organizing Without Organizations by Clay Shirky
- The Tao of Programming
- Computational Beauty of Nature
- Writing Solid Code by Steve Maguire
- Philip and Alex's Guide to Web Publishing
- Object-Oriented Analysis and Design with Applications by Grady Booch
- Effective Java by Joshua Bloch
- Computability by N. J. Cutland
- Masterminds of Programming
- The Tao Te Ching
- The Productive Programmer
- The Art of Deception by Kevin Mitnick
- The Career Programmer: Guerilla Tactics for an Imperfect World by Christopher Duncan
- Paradigms of Artificial Intelligence Programming: Case studies in Common Lisp
- Masters of Doom
- Pragmatic Unit Testing in C# with NUnit by Andy Hunt and Dave Thomas with Matt Hargett
- How To Solve It by George Polya
- The Alchemist by Paulo Coelho
- Smalltalk-80: The Language and its Implementation
- Writing Secure Code (2nd Edition) by Michael Howard
- Introduction to Functional Programming by Philip Wadler and Richard Bird
- No Bugs! by David Thielen
- Rework by Jason Freid and DHH
- JUnit in Action
#BlackOwned #BlackEntrepreneurs #BlackBuniness #AWSCertified #AWSCloudPractitioner #AWSCertification #AWSCLFC02 #CloudComputing #AWSStudyGuide #AWSTraining #AWSCareer #AWSExamPrep #AWSCommunity #AWSEducation #AWSBasics #AWSCertified #AWSMachineLearning #AWSCertification #AWSSpecialty #MachineLearning #AWSStudyGuide #CloudComputing #DataScience #AWSCertified #AWSSolutionsArchitect #AWSArchitectAssociate #AWSCertification #AWSStudyGuide #CloudComputing #AWSArchitecture #AWSTraining #AWSCareer #AWSExamPrep #AWSCommunity #AWSEducation #AzureFundamentals #AZ900 #MicrosoftAzure #ITCertification #CertificationPrep #StudyMaterials #TechLearning #MicrosoftCertified #AzureCertification #TechBooks
Top 1000 Canada Quiz and trivia: CANADA CITIZENSHIP TEST- HISTORY - GEOGRAPHY - GOVERNMENT- CULTURE - PEOPLE - LANGUAGES - TRAVEL - WILDLIFE - HOCKEY - TOURISM - SCENERIES - ARTS - DATA VISUALIZATION

Top 1000 Africa Quiz and trivia: HISTORY - GEOGRAPHY - WILDLIFE - CULTURE - PEOPLE - LANGUAGES - TRAVEL - TOURISM - SCENERIES - ARTS - DATA VISUALIZATION

Exploring the Pros and Cons of Visiting All Provinces and Territories in Canada.

Exploring the Advantages and Disadvantages of Visiting All 50 States in the USA

Health Health, a science-based community to discuss health news and the coronavirus (COVID-19) pandemic
- Two US vaccines could ship in weeks if bird flu jumps from infected cows to humansby /u/lurker_bee on May 2, 2024 at 11:05 pm
submitted by /u/lurker_bee [link] [comments]
- Help Wisconsin Watch report on long-term care in the stateby /u/jms1225 on May 2, 2024 at 10:00 pm
submitted by /u/jms1225 [link] [comments]
- The information problems around the Andrew Huberman podcastby /u/mcgillhufflepuff on May 2, 2024 at 8:32 pm
submitted by /u/mcgillhufflepuff [link] [comments]
- A new form of mpox that may spread more easily found in Congo's biggest outbreakby /u/Exastiken on May 2, 2024 at 7:50 pm
submitted by /u/Exastiken [link] [comments]
- It may be safe for some to wait 15 years for repeat colonoscopy, study suggestsby /u/nbcnews on May 2, 2024 at 7:08 pm
submitted by /u/nbcnews [link] [comments]
Today I Learned (TIL) You learn something new every day; what did you learn today? Submit interesting and specific facts about something that you just found out here.
- TIL John Von Neumann worked on the first atomic bomb and the first computer, came up with the formulas for quantum mechanics, described genetic self-replication before the discovery of DNA, and founded the field of game theory, among other things. He has often been called the smartest man ever.by /u/HumanNutrStudent on May 3, 2024 at 2:46 am
submitted by /u/HumanNutrStudent [link] [comments]
- TIL: The Rocko's Modern Life theme song was preform by the B-52's and Tom Kenny (the voice of SpongeBob)by /u/Material-Apple1289 on May 3, 2024 at 2:42 am
submitted by /u/Material-Apple1289 [link] [comments]
- TIL that after the plane crash in which Buddy Holly died, the remaining members of his band continued the tourby /u/A_Mirabeau_702 on May 3, 2024 at 12:25 am
submitted by /u/A_Mirabeau_702 [link] [comments]
- TIL the FBI has an entire catalogue of times they witnessed and recorded UFOs.by /u/Lapwing1916 on May 3, 2024 at 12:21 am
submitted by /u/Lapwing1916 [link] [comments]
- TIL that actress Alicia Witt's parents were found frozen to death in their Massachusetts home in December 2021.They refused help on home repairs repeatedly.by /u/Cultural_Magician105 on May 3, 2024 at 12:17 am
submitted by /u/Cultural_Magician105 [link] [comments]
Reddit Science This community is a place to share and discuss new scientific research. Read about the latest advances in astronomy, biology, medicine, physics, social science, and more. Find and submit new publications and popular science coverage of current research.
- Physician Antipsychotic Overprescribing Letters and Outcomes Among People With Dementiaby /u/burtzev on May 2, 2024 at 11:46 pm
submitted by /u/burtzev [link] [comments]
- Poverty tops smoking as a major death risk in the US : A study found that Black and white people who earned less than $15,000 a year died, on average, more than 10 years earlier than those whose annual income exceeded $50,000.by /u/Wagamaga on May 2, 2024 at 6:22 pm
submitted by /u/Wagamaga [link] [comments]
- Study suggests hero label may lead to exploitation of nurses, teachers, and military. Heroization intends to honor and motivate, highlighting altruism and sacrifice. However, when people view them as inherently self-sacrificing, they may be less responsive to exploitation faced by these individuals.by /u/mvea on May 2, 2024 at 6:19 pm
submitted by /u/mvea [link] [comments]
- New findings point to an Earth-like environment on ancient Mars - Manganese-rich sandstones indicate there were once habitable conditions in the Gale Craterby /u/GeoGeoGeoGeo on May 2, 2024 at 5:58 pm
submitted by /u/GeoGeoGeoGeo [link] [comments]
- Intel researchers demonstrated a cryogenic probing technique for automated characterization of silicon qubit cells enabling significantly faster feedback leading to higher yields and lower process variationby /u/catch878 on May 2, 2024 at 5:28 pm
submitted by /u/catch878 [link] [comments]
Reddit Sports Sports News and Highlights from the NFL, NBA, NHL, MLB, MLS, and leagues around the world.
- Knicks outlast 76ers in Game 6 to win thrilling seriesby /u/Oldtimer_2 on May 3, 2024 at 3:47 am
submitted by /u/Oldtimer_2 [link] [comments]
- Beverley chucks ball at Pacers fans twice during Bucks' lossby /u/Oldtimer_2 on May 3, 2024 at 3:03 am
submitted by /u/Oldtimer_2 [link] [comments]
- Bruins outplayed by Maple Leafs in Game 6, setting up Game 7 in Bostonby /u/Oldtimer_2 on May 3, 2024 at 2:59 am
submitted by /u/Oldtimer_2 [link] [comments]
- Paris inaugurates River Seine water storage for Olympic swimmingby /u/PrincessBananas85 on May 3, 2024 at 2:01 am
submitted by /u/PrincessBananas85 [link] [comments]
- Pacers' team effort eliminates Bucks in Game 6by /u/Oldtimer_2 on May 3, 2024 at 1:10 am
submitted by /u/Oldtimer_2 [link] [comments]