Decoding GPTs & LLMs: Training, Memory & Advanced Architectures Explained
Unlock the secrets of GPTs and Large Language Models (LLMs) in our comprehensive guide!
🤖🚀 Dive deep into the world of AI as we explore ‘GPTs and LLMs: Pre-Training, Fine-Tuning, Memory, and More!’ Understand the intricacies of how these AI models learn through pre-training and fine-tuning, their operational scope within a context window, and the intriguing aspect of their lack of long-term memory.
🧠 In this article, we demystify:
- Pre-Training & Fine-Tuning Methods: Learn how GPTs and LLMs are trained on vast datasets to grasp language patterns and how fine-tuning tailors them for specific tasks.
- Context Window in AI: Explore the concept of the context window, which acts as a short-term memory for LLMs, influencing how they process and respond to information.
- Lack of Long-Term Memory: Understand the limitations of GPTs and LLMs in retaining information over extended periods and how this impacts their functionality.
- Database-Querying Architectures: Discover how some advanced AI models interact with external databases to enhance information retrieval and processing.
- PDF Apps & Real-Time Fine-Tuning
Drop your questions and thoughts in the comments below and let’s discuss the future of AI! #GPTsExplained #LLMs #AITraining #MachineLearning #AIContextWindow #AILongTermMemory #AIDatabases #PDFAppsAI”
Subscribe for weekly updates and deep dives into artificial intelligence innovations.
✅ Don’t forget to Like, Comment, and Share this video to support our content.
📌 Check out our playlist for more AI insights
📖 Read along with the podcast below:
Welcome to AI Unraveled, the podcast that demystifies frequently asked questions on artificial intelligence and keeps you up to date with the latest AI trends. Join us as we delve into groundbreaking research, innovative applications, and emerging technologies that are pushing the boundaries of AI. From the latest trends in ChatGPT and the recent merger of Google Brain and DeepMind, to the exciting developments in generative AI, we’ve got you covered with a comprehensive update on the ever-evolving AI landscape. In today’s episode, we’ll cover GPTs and LLMs, their pre-training and fine-tuning methods, their context window and lack of long-term memory, architectures that query databases, PDF app’s use of near-realtime fine-tuning, and the book “AI Unraveled” which answers FAQs about AI.
GPTs, or Generative Pre-trained Transformers, work by being trained on a large amount of text data and then using that training to generate output based on input. So, when you give a GPT a specific input, it will produce the best matching output based on its training.
The way GPTs do this is by processing the input token by token, without actually understanding the entire output. It simply recognizes that certain tokens are often followed by certain other tokens based on its training. This knowledge is gained during the training process, where the language model (LLM) is fed a large number of embeddings, which can be thought of as its “knowledge.”
After the training stage, a LLM can be fine-tuned to improve its accuracy for a particular domain. This is done by providing it with domain-specific labeled data and modifying its parameters to match the desired accuracy on that data.
Now, let’s talk about “memory” in these models. LLMs do not have a long-term memory in the same way humans do. If you were to tell an LLM that you have a 6-year-old son, it wouldn’t retain that information like a human would. However, these models can still answer related follow-up questions in a conversation.
Advertise with us - Post Your Good Content Here
We are ranked in the Top 20 on Google
AI Dashboard is available on the Web, Apple, Google, and Microsoft, PRO version
For example, if you ask the model to tell you a story and then ask it to make the story shorter, it can generate a shorter version of the story. This is possible because the previous Q&A is passed along in the context window of the conversation. The context window keeps track of the conversation history, allowing the model to maintain some context and generate appropriate responses.
As the conversation continues, the context window and the number of tokens required will keep growing. This can become a challenge, as there are limitations on the maximum length of input that the model can handle. If a conversation becomes too long, the model may start truncating or forgetting earlier parts of the conversation.
Regarding architectures and databases, there are some models that may query a database before providing an answer. For example, a model could be designed to run a database query like “select * from user_history” to retrieve relevant information before generating a response. This is one way vector databases can be used in the context of these models.
There are also architectures where the model undergoes near-realtime fine-tuning when a chat begins. This means that the model is fine-tuned on specific data related to the chat session itself, which helps it generate more context-aware responses. This is similar to how “speak with your PDF” apps work, where the model is trained on specific PDF content to provide relevant responses.
In summary, GPTs and LLMs work by being pre-trained on a large amount of text data and then using that training to generate output based on input. They do this token by token, without truly understanding the complete output. LLMs can be fine-tuned to improve accuracy for specific domains by providing them with domain-specific labeled data. While LLMs don’t have long-term memory like humans, they can still generate responses in a conversation by using the context window to keep track of the conversation history. Some architectures may query databases before generating responses, and others may undergo near-realtime fine-tuning to provide more context-aware answers.
GPTs and Large Language Models (LLMs) are fascinating tools that have revolutionized natural language processing. It seems like you have a good grasp of how these models function, but I’ll take a moment to provide some clarification and expand on a few points for a more comprehensive understanding.
When it comes to GPTs and LLMs, pre-training and token prediction play a crucial role. During the pre-training phase, these models are exposed to massive amounts of text data. This helps them learn to predict the next token (word or part of a word) in a sequence based on the statistical likelihood of that token following the given context. It’s important to note that while the model can recognize patterns in language use, it doesn’t truly “understand” the text in a human sense.
During the training process, the model becomes familiar with these large datasets and learns embeddings. Embeddings are representations of tokens in a high-dimensional space, and they capture relationships and context around each token. These embeddings allow the model to generate coherent and contextually appropriate responses.
However, pre-training is just the beginning. Fine-tuning is a subsequent step that tailors the model to specific domains or tasks. It involves training the model further on a smaller, domain-specific dataset. This process adjusts the model’s parameters, enabling it to generate responses that are more relevant to the specialized domain.
Now, let’s discuss memory and the context window. LLMs like GPT do not possess long-term memory in the same way humans do. Instead, they operate within what we call a context window. The context window determines the amount of text (measured in tokens) that the model can consider when making predictions. It provides the model with a form of “short-term memory.”
For follow-up questions, the model relies on this context window. So, when you ask a follow-up question, the model factors in the previous interaction (the original story and the request to shorten it) within its context window. It then generates a response based on that context. However, it’s crucial to note that the context window has a fixed size, which means it can only hold a certain number of tokens. If the conversation exceeds this limit, the oldest tokens are discarded, and the model loses track of that part of the dialogue.
It’s also worth mentioning that there is no real-time fine-tuning happening with each interaction. The model responds based on its pre-training and any fine-tuning that occurred prior to its deployment. This means that the model does not learn or adapt during real-time conversation but rather relies on the knowledge it has gained from pre-training and fine-tuning.
While standard LLMs like GPT do not typically utilize external memory systems or databases, some advanced models and applications may incorporate these features. External memory systems can store information beyond the limits of the context window. However, it’s important to understand that these features are not inherent to the base LLM architecture like GPT. In some systems, vector databases might be used to enhance the retrieval of relevant information based on queries, but this is separate from the internal processing of the LLM.
In relation to the “speak with your PDF” applications you mentioned, they generally employ a combination of text extraction and LLMs. The purpose is to interpret and respond to queries about the content of a PDF. These applications do not engage in real-time fine-tuning, but instead use the existing capabilities of the model to interpret and interact with the newly extracted text.
To summarize, LLMs like GPT operate within a context window and utilize patterns learned during pre-training and fine-tuning to generate responses. They do not possess long-term memory or real-time learning capabilities during interactions, but they can handle follow-up questions within the confines of their context window. It’s important to remember that while some advanced implementations might leverage external memory or databases, these features are not inherently built into the foundational architecture of the standard LLM.
Are you ready to dive into the fascinating world of artificial intelligence? Well, I’ve got just the thing for you! It’s an incredible book called “AI Unraveled: Demystifying Frequently Asked Questions on Artificial Intelligence.” Trust me, this book is an absolute gem!
Now, you might be wondering where you can get your hands on this treasure trove of knowledge. Look no further, my friend. You can find “AI Unraveled” at popular online platforms like Etsy, Shopify, Apple, Google, and of course, our old faithful, Amazon.
This book is a must-have for anyone eager to expand their understanding of AI. It takes those complicated concepts and breaks them down into easily digestible chunks. No more scratching your head in confusion or getting lost in a sea of technical terms. With “AI Unraveled,” you’ll gain a clear and concise understanding of artificial intelligence.
So, if you’re ready to embark on this incredible journey of unraveling the mysteries of AI, go ahead and grab your copy of “AI Unraveled” today. Trust me, you won’t regret it!
On today’s episode, we explored the power of GPTs and LLMs, discussing their ability to generate outputs, be fine-tuned for specific domains, and utilize a context window for related follow-up questions. We also learned about their limitations in terms of long-term memory and real-time updates. Lastly, we shared information about the book “AI Unraveled,” which provides valuable insights into the world of artificial intelligence. Join us next time on AI Unraveled as we continue to demystify frequently asked questions on artificial intelligence and bring you the latest trends in AI, including ChatGPT advancements and the exciting collaboration between Google Brain and DeepMind. Stay informed, stay curious, and don’t forget to subscribe for more!
📢 Advertise with us and Sponsorship Opportunities
Are you eager to expand your understanding of artificial intelligence? Look no further than the essential book “AI Unraveled: Demystifying Frequently Asked Questions on Artificial Intelligence,” available at Etsy, Shopify, Apple, Google, or Amazon

- AI can't take people's jobs if there's no people.by /u/baalzimon (Artificial Intelligence Gateway) on July 26, 2024 at 10:53 am
Looks more and more likely that human populations will decline in the future. Maybe the workforce will just be AI robots rather than young people. PEW: The Experiences of U.S. Adults Who Don’t Have Children 57% of adults under 50 who say they’re unlikely to ever have kids say a major reason is they just don’t want to; 31% of those ages 50 and older without kids cite this as a reason they never had them https://www.pewresearch.org/social-trends/2024/07/25/the-experiences-of-u-s-adults-who-dont-have-children/ submitted by /u/baalzimon [link] [comments]
- UK School Under Fire for Unlawful Facial-Recognition Useby /u/Think_Cat1101 (Artificial Intelligence Gateway) on July 26, 2024 at 10:43 am
https://www.msn.com/en-us/news/technology/uk-school-under-fire-for-unlawful-facial-recognition-use/ar-BB1qEmeX?cvid=6dfe65854c6e4c2ad473b0e649e795b2&ei=10 submitted by /u/Think_Cat1101 [link] [comments]
- OpenAI reveals 'SearchGPT'by /u/Mindful-AI (Artificial Intelligence Gateway) on July 26, 2024 at 10:41 am
submitted by /u/Mindful-AI [link] [comments]
- Amazon’s AI Chip Revolution: How They’re Ditching Nvidia’s High Prices and Speeding Aheadby /u/alyis4u (Artificial Intelligence Gateway) on July 26, 2024 at 9:23 am
Six engineers tested a brand-new, secret server design on a Friday afternoon in Amazon.com’s chip lab in Austin, Texas. Amazon executive Rami Sinno said on Friday during a visit to the lab that the server was full of Amazon’s AI chips, which compete with Nvidia’s chips and are the market leader.https://theaiwired.com/amazons-ai-chip-revolution-how-theyre-ditching-nvidias-high-prices-and-speeding-ahead/ submitted by /u/alyis4u [link] [comments]
- OpenAI's SearchGPT Is Coming For Google Search; Here Are The Features That Will Reportedly Make It Betterby /u/vinaylovestotravel (Artificial Intelligence Gateway) on July 26, 2024 at 9:00 am
Dubbed "SearchGPT," the tool will offer "fast and timely answers with clear and relevant sources" by referencing content from websites and news publishers, including OpenAI content partners such as News Corp (The Post's parent company) and The Atlantic. Read more: https://www.ibtimes.co.uk/openais-searchgpt-coming-google-search-here-are-features-that-will-reportedly-make-it-better-1725770 submitted by /u/vinaylovestotravel [link] [comments]
- Deleting chats from Blackbox AI?by /u/Intelligent-Fig-7791 (Artificial Intelligence Gateway) on July 26, 2024 at 7:40 am
How on earth do you delete chats from blackbox.ai ? it seems like all chats are public by default submitted by /u/Intelligent-Fig-7791 [link] [comments]
- Creating Excel files from tricky PDFs with Google's new multimodal AI (Gemini Flash)by /u/Confident-Honeydew66 (Artificial Intelligence Gateway) on July 26, 2024 at 6:42 am
I wrote a guide on how I use Google's new AI model (gemini flash) to extract structured data, ready for excel, from the most visually complex of PDFs. I figure this AI crowd may be interested. The key points I cover are: Defining perfect prompts using schemas for data extraction Using Google gemini's multimodal capabilities for PDF parsing Exporting results to XLSX or CSV Here's the guide for anyone interested! submitted by /u/Confident-Honeydew66 [link] [comments]
- Tech stocks fall in US and Asia as AI shares declineby /u/farooqui45 (Artificial Intelligence Gateway) on July 26, 2024 at 4:12 am
Tech prices fell sharply in both the US and Asia. Shares of AI companies fell the most, which hurt big companies. The US and Asian stock markets have dropped sharply as buyers sell off their shares in tech companies. Stocks in artificial intelligence (AI) companies have been hit the hardest.Read More Here. submitted by /u/farooqui45 [link] [comments]
- One-Minute Daily AI News 7/25/2024by /u/Excellent-Target-847 (Artificial Intelligence Gateway) on July 26, 2024 at 3:20 am
OpenAI announces SearchGPT, its AI-powered search engine.[1] Lawmaker uses AI voice clone to address Congress.[2] Announcing Phi-3 fine-tuning, new generative AI models, and other Azure AI updates to empower organizations to customize and scale AI applications.[3] Runway’s AI video generator trained on thousands of scraped YouTube videos.[4] Sources included at: https://bushaicave.com/2024/07/25/7-25-2024/ submitted by /u/Excellent-Target-847 [link] [comments]
- Guidance on AI Engine/Dataflow Designby /u/realeyezayuh (Artificial Intelligence Gateway) on July 26, 2024 at 2:58 am
Hello, Community, I work for a medium sized organization that is on Azure cloud for most all our services. Our software team is like a startup where we have four guys doing a lot of the in house software dev for our organization - mainly on the cloud (Azure). A problem we are trying to solve is to generate some sort of process that will take in historical data --> categorize the data given a category list dataset ---> spit out a "trained dataset". As new data comes in it would replace the historical data and come in through the workflow above and categorize new data coming in. What is the best method/route to explore in Azure to make this happen end to end? I appreciate your help in advance and will gladly answer any questions you might have in addition to gain more insight. submitted by /u/realeyezayuh [link] [comments]
- AI can't take people's jobs if there's no people.by /u/baalzimon (Artificial Intelligence Gateway) on July 26, 2024 at 10:53 am
Looks more and more likely that human populations will decline in the future. Maybe the workforce will just be AI robots rather than young people. PEW: The Experiences of U.S. Adults Who Don’t Have Children 57% of adults under 50 who say they’re unlikely to ever have kids say a major reason is they just don’t want to; 31% of those ages 50 and older without kids cite this as a reason they never had them https://www.pewresearch.org/social-trends/2024/07/25/the-experiences-of-u-s-adults-who-dont-have-children/ submitted by /u/baalzimon [link] [comments]
- UK School Under Fire for Unlawful Facial-Recognition Useby /u/Think_Cat1101 (Artificial Intelligence Gateway) on July 26, 2024 at 10:43 am
https://www.msn.com/en-us/news/technology/uk-school-under-fire-for-unlawful-facial-recognition-use/ar-BB1qEmeX?cvid=6dfe65854c6e4c2ad473b0e649e795b2&ei=10 submitted by /u/Think_Cat1101 [link] [comments]
- OpenAI reveals 'SearchGPT'by /u/Mindful-AI (Artificial Intelligence Gateway) on July 26, 2024 at 10:41 am
submitted by /u/Mindful-AI [link] [comments]
- Amazon’s AI Chip Revolution: How They’re Ditching Nvidia’s High Prices and Speeding Aheadby /u/alyis4u (Artificial Intelligence Gateway) on July 26, 2024 at 9:23 am
Six engineers tested a brand-new, secret server design on a Friday afternoon in Amazon.com’s chip lab in Austin, Texas. Amazon executive Rami Sinno said on Friday during a visit to the lab that the server was full of Amazon’s AI chips, which compete with Nvidia’s chips and are the market leader.https://theaiwired.com/amazons-ai-chip-revolution-how-theyre-ditching-nvidias-high-prices-and-speeding-ahead/ submitted by /u/alyis4u [link] [comments]
- OpenAI's SearchGPT Is Coming For Google Search; Here Are The Features That Will Reportedly Make It Betterby /u/vinaylovestotravel (Artificial Intelligence Gateway) on July 26, 2024 at 9:00 am
Dubbed "SearchGPT," the tool will offer "fast and timely answers with clear and relevant sources" by referencing content from websites and news publishers, including OpenAI content partners such as News Corp (The Post's parent company) and The Atlantic. Read more: https://www.ibtimes.co.uk/openais-searchgpt-coming-google-search-here-are-features-that-will-reportedly-make-it-better-1725770 submitted by /u/vinaylovestotravel [link] [comments]
- Deleting chats from Blackbox AI?by /u/Intelligent-Fig-7791 (Artificial Intelligence Gateway) on July 26, 2024 at 7:40 am
How on earth do you delete chats from blackbox.ai ? it seems like all chats are public by default submitted by /u/Intelligent-Fig-7791 [link] [comments]
- Creating Excel files from tricky PDFs with Google's new multimodal AI (Gemini Flash)by /u/Confident-Honeydew66 (Artificial Intelligence Gateway) on July 26, 2024 at 6:42 am
I wrote a guide on how I use Google's new AI model (gemini flash) to extract structured data, ready for excel, from the most visually complex of PDFs. I figure this AI crowd may be interested. The key points I cover are: Defining perfect prompts using schemas for data extraction Using Google gemini's multimodal capabilities for PDF parsing Exporting results to XLSX or CSV Here's the guide for anyone interested! submitted by /u/Confident-Honeydew66 [link] [comments]
- Tech stocks fall in US and Asia as AI shares declineby /u/farooqui45 (Artificial Intelligence Gateway) on July 26, 2024 at 4:12 am
Tech prices fell sharply in both the US and Asia. Shares of AI companies fell the most, which hurt big companies. The US and Asian stock markets have dropped sharply as buyers sell off their shares in tech companies. Stocks in artificial intelligence (AI) companies have been hit the hardest.Read More Here. submitted by /u/farooqui45 [link] [comments]
- One-Minute Daily AI News 7/25/2024by /u/Excellent-Target-847 (Artificial Intelligence Gateway) on July 26, 2024 at 3:20 am
OpenAI announces SearchGPT, its AI-powered search engine.[1] Lawmaker uses AI voice clone to address Congress.[2] Announcing Phi-3 fine-tuning, new generative AI models, and other Azure AI updates to empower organizations to customize and scale AI applications.[3] Runway’s AI video generator trained on thousands of scraped YouTube videos.[4] Sources included at: https://bushaicave.com/2024/07/25/7-25-2024/ submitted by /u/Excellent-Target-847 [link] [comments]
- Guidance on AI Engine/Dataflow Designby /u/realeyezayuh (Artificial Intelligence Gateway) on July 26, 2024 at 2:58 am
Hello, Community, I work for a medium sized organization that is on Azure cloud for most all our services. Our software team is like a startup where we have four guys doing a lot of the in house software dev for our organization - mainly on the cloud (Azure). A problem we are trying to solve is to generate some sort of process that will take in historical data --> categorize the data given a category list dataset ---> spit out a "trained dataset". As new data comes in it would replace the historical data and come in through the workflow above and categorize new data coming in. What is the best method/route to explore in Azure to make this happen end to end? I appreciate your help in advance and will gladly answer any questions you might have in addition to gain more insight. submitted by /u/realeyezayuh [link] [comments]
Active Hydrating Toner, Anti-Aging Replenishing Advanced Face Moisturizer, with Vitamins A, C, E & Natural Botanicals to Promote Skin Balance & Collagen Production, 6.7 Fl Oz
Age Defying 0.3% Retinol Serum, Anti-Aging Dark Spot Remover for Face, Fine Lines & Wrinkle Pore Minimizer, with Vitamin E & Natural Botanicals
Firming Moisturizer, Advanced Hydrating Facial Replenishing Cream, with Hyaluronic Acid, Resveratrol & Natural Botanicals to Restore Skin's Strength, Radiance, and Resilience, 1.75 Oz
Skin Stem Cell Serum
Smartphone 101 - Pick a smartphone for me - android or iOS - Apple iPhone or Samsung Galaxy or Huawei or Xaomi or Google Pixel
Can AI Really Predict Lottery Results? We Asked an Expert.
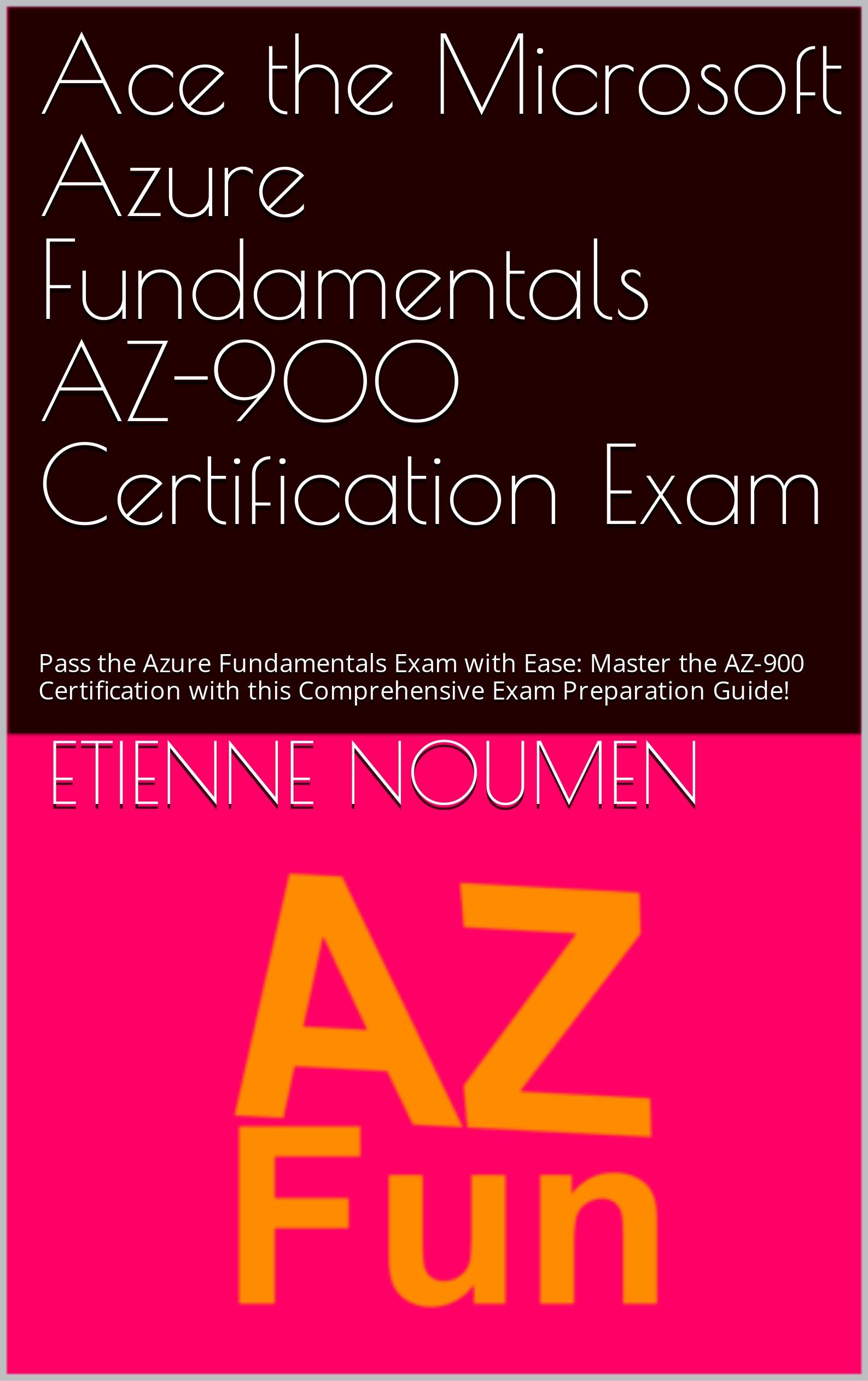

Djamgatech

Read Photos and PDFs Aloud for me iOS
Read Photos and PDFs Aloud for me android
Read Photos and PDFs Aloud For me Windows 10/11
Read Photos and PDFs Aloud For Amazon
Get 20% off Google Workspace (Google Meet) Business Plan (AMERICAS): M9HNXHX3WC9H7YE (Email us for more)
Get 20% off Google Google Workspace (Google Meet) Standard Plan with the following codes: 96DRHDRA9J7GTN6(Email us for more)
FREE 10000+ Quiz Trivia and and Brain Teasers for All Topics including Cloud Computing, General Knowledge, History, Television, Music, Art, Science, Movies, Films, US History, Soccer Football, World Cup, Data Science, Machine Learning, Geography, etc....

List of Freely available programming books - What is the single most influential book every Programmers should read
- Bjarne Stroustrup - The C++ Programming Language
- Brian W. Kernighan, Rob Pike - The Practice of Programming
- Donald Knuth - The Art of Computer Programming
- Ellen Ullman - Close to the Machine
- Ellis Horowitz - Fundamentals of Computer Algorithms
- Eric Raymond - The Art of Unix Programming
- Gerald M. Weinberg - The Psychology of Computer Programming
- James Gosling - The Java Programming Language
- Joel Spolsky - The Best Software Writing I
- Keith Curtis - After the Software Wars
- Richard M. Stallman - Free Software, Free Society
- Richard P. Gabriel - Patterns of Software
- Richard P. Gabriel - Innovation Happens Elsewhere
- Code Complete (2nd edition) by Steve McConnell
- The Pragmatic Programmer
- Structure and Interpretation of Computer Programs
- The C Programming Language by Kernighan and Ritchie
- Introduction to Algorithms by Cormen, Leiserson, Rivest & Stein
- Design Patterns by the Gang of Four
- Refactoring: Improving the Design of Existing Code
- The Mythical Man Month
- The Art of Computer Programming by Donald Knuth
- Compilers: Principles, Techniques and Tools by Alfred V. Aho, Ravi Sethi and Jeffrey D. Ullman
- Gödel, Escher, Bach by Douglas Hofstadter
- Clean Code: A Handbook of Agile Software Craftsmanship by Robert C. Martin
- Effective C++
- More Effective C++
- CODE by Charles Petzold
- Programming Pearls by Jon Bentley
- Working Effectively with Legacy Code by Michael C. Feathers
- Peopleware by Demarco and Lister
- Coders at Work by Peter Seibel
- Surely You're Joking, Mr. Feynman!
- Effective Java 2nd edition
- Patterns of Enterprise Application Architecture by Martin Fowler
- The Little Schemer
- The Seasoned Schemer
- Why's (Poignant) Guide to Ruby
- The Inmates Are Running The Asylum: Why High Tech Products Drive Us Crazy and How to Restore the Sanity
- The Art of Unix Programming
- Test-Driven Development: By Example by Kent Beck
- Practices of an Agile Developer
- Don't Make Me Think
- Agile Software Development, Principles, Patterns, and Practices by Robert C. Martin
- Domain Driven Designs by Eric Evans
- The Design of Everyday Things by Donald Norman
- Modern C++ Design by Andrei Alexandrescu
- Best Software Writing I by Joel Spolsky
- The Practice of Programming by Kernighan and Pike
- Pragmatic Thinking and Learning: Refactor Your Wetware by Andy Hunt
- Software Estimation: Demystifying the Black Art by Steve McConnel
- The Passionate Programmer (My Job Went To India) by Chad Fowler
- Hackers: Heroes of the Computer Revolution
- Algorithms + Data Structures = Programs
- Writing Solid Code
- JavaScript - The Good Parts
- Getting Real by 37 Signals
- Foundations of Programming by Karl Seguin
- Computer Graphics: Principles and Practice in C (2nd Edition)
- Thinking in Java by Bruce Eckel
- The Elements of Computing Systems
- Refactoring to Patterns by Joshua Kerievsky
- Modern Operating Systems by Andrew S. Tanenbaum
- The Annotated Turing
- Things That Make Us Smart by Donald Norman
- The Timeless Way of Building by Christopher Alexander
- The Deadline: A Novel About Project Management by Tom DeMarco
- The C++ Programming Language (3rd edition) by Stroustrup
- Patterns of Enterprise Application Architecture
- Computer Systems - A Programmer's Perspective
- Agile Principles, Patterns, and Practices in C# by Robert C. Martin
- Growing Object-Oriented Software, Guided by Tests
- Framework Design Guidelines by Brad Abrams
- Object Thinking by Dr. David West
- Advanced Programming in the UNIX Environment by W. Richard Stevens
- Hackers and Painters: Big Ideas from the Computer Age
- The Soul of a New Machine by Tracy Kidder
- CLR via C# by Jeffrey Richter
- The Timeless Way of Building by Christopher Alexander
- Design Patterns in C# by Steve Metsker
- Alice in Wonderland by Lewis Carol
- Zen and the Art of Motorcycle Maintenance by Robert M. Pirsig
- About Face - The Essentials of Interaction Design
- Here Comes Everybody: The Power of Organizing Without Organizations by Clay Shirky
- The Tao of Programming
- Computational Beauty of Nature
- Writing Solid Code by Steve Maguire
- Philip and Alex's Guide to Web Publishing
- Object-Oriented Analysis and Design with Applications by Grady Booch
- Effective Java by Joshua Bloch
- Computability by N. J. Cutland
- Masterminds of Programming
- The Tao Te Ching
- The Productive Programmer
- The Art of Deception by Kevin Mitnick
- The Career Programmer: Guerilla Tactics for an Imperfect World by Christopher Duncan
- Paradigms of Artificial Intelligence Programming: Case studies in Common Lisp
- Masters of Doom
- Pragmatic Unit Testing in C# with NUnit by Andy Hunt and Dave Thomas with Matt Hargett
- How To Solve It by George Polya
- The Alchemist by Paulo Coelho
- Smalltalk-80: The Language and its Implementation
- Writing Secure Code (2nd Edition) by Michael Howard
- Introduction to Functional Programming by Philip Wadler and Richard Bird
- No Bugs! by David Thielen
- Rework by Jason Freid and DHH
- JUnit in Action
#BlackOwned #BlackEntrepreneurs #BlackBuniness #AWSCertified #AWSCloudPractitioner #AWSCertification #AWSCLFC02 #CloudComputing #AWSStudyGuide #AWSTraining #AWSCareer #AWSExamPrep #AWSCommunity #AWSEducation #AWSBasics #AWSCertified #AWSMachineLearning #AWSCertification #AWSSpecialty #MachineLearning #AWSStudyGuide #CloudComputing #DataScience #AWSCertified #AWSSolutionsArchitect #AWSArchitectAssociate #AWSCertification #AWSStudyGuide #CloudComputing #AWSArchitecture #AWSTraining #AWSCareer #AWSExamPrep #AWSCommunity #AWSEducation #AzureFundamentals #AZ900 #MicrosoftAzure #ITCertification #CertificationPrep #StudyMaterials #TechLearning #MicrosoftCertified #AzureCertification #TechBooks
Top 1000 Canada Quiz and trivia: CANADA CITIZENSHIP TEST- HISTORY - GEOGRAPHY - GOVERNMENT- CULTURE - PEOPLE - LANGUAGES - TRAVEL - WILDLIFE - HOCKEY - TOURISM - SCENERIES - ARTS - DATA VISUALIZATION

Top 1000 Africa Quiz and trivia: HISTORY - GEOGRAPHY - WILDLIFE - CULTURE - PEOPLE - LANGUAGES - TRAVEL - TOURISM - SCENERIES - ARTS - DATA VISUALIZATION

Exploring the Pros and Cons of Visiting All Provinces and Territories in Canada.

Exploring the Advantages and Disadvantages of Visiting All 50 States in the USA

Health Health, a science-based community to discuss health news and the coronavirus (COVID-19) pandemic
- The pull-out method: Why this common contraceptive fails to deliverby /u/Kampala_Dispatch on July 26, 2024 at 7:51 pm
submitted by /u/Kampala_Dispatch [link] [comments]
- Health Canada data reveals surprising number of adverse cannabis reactions (spoiler: it's small)by /u/carajuana_readit on July 26, 2024 at 5:49 pm
submitted by /u/carajuana_readit [link] [comments]
- Online portals deliver scary health news before doctors can weigh inby /u/washingtonpost on July 26, 2024 at 4:37 pm
submitted by /u/washingtonpost [link] [comments]
- Vaccine 'sharply cuts risk of dementia' new study findsby /u/SubstantialSnow7114 on July 26, 2024 at 1:53 pm
submitted by /u/SubstantialSnow7114 [link] [comments]
- Calls to limit sexual partners as mpox makes a resurgence in Australiaby /u/boppinmule on July 26, 2024 at 12:31 pm
submitted by /u/boppinmule [link] [comments]
Today I Learned (TIL) You learn something new every day; what did you learn today? Submit interesting and specific facts about something that you just found out here.
- TIL that in Thailand, if your spouse cheats on you, you can legally sue their lover for damages and can receive up to 5,000,000 THB ($140,000 USD) or more under Section 1523 of the Thai Civil and Commercial Codeby /u/Mavrokordato on July 26, 2024 at 6:57 pm
submitted by /u/Mavrokordato [link] [comments]
- TIL that with a population of 170 million people, Bangladesh is the most populous country to have never won a medal at the Olympic Games.by /u/Blackraven2007 on July 26, 2024 at 6:49 pm
submitted by /u/Blackraven2007 [link] [comments]
- TIL a psychologist got himself admitted to a mental hospital by claiming he heard the words "empty", "hollow" and "thud" in his head. Then, it took him two months to convince them he was sane, after agreeing he was insane and accepting medication.by /u/Hadeverse-050 on July 26, 2024 at 6:44 pm
submitted by /u/Hadeverse-050 [link] [comments]
- TIL Senator John Edwards of NC, USA cheated on his wife and had a child with another woman. He tried to deny it but eventually caved and admitted his mistake. He used campaign funds and was indicted by a grand jury. His life story inspired the show "The Good Wife" by Robert & Michelle Kingby /u/AdvisorPast637 on July 26, 2024 at 6:09 pm
submitted by /u/AdvisorPast637 [link] [comments]
- TIL Zhang Shuhong was a Chinese businessman who committed suicide after toys made at his factory for Fisher-Price (a division of Mattel) were found to contain lead paintby /u/Hopeful-Candle-4884 on July 26, 2024 at 4:43 pm
submitted by /u/Hopeful-Candle-4884 [link] [comments]
Reddit Science This community is a place to share and discuss new scientific research. Read about the latest advances in astronomy, biology, medicine, physics, social science, and more. Find and submit new publications and popular science coverage of current research.
- Human decision makers who possess the authority to override ML predictions may impede the self-correction of discriminatory models and even induce initially unbiased models to become discriminatory with timeby /u/f1u82ypd on July 26, 2024 at 6:29 pm
submitted by /u/f1u82ypd [link] [comments]
- Study uses Game of Thrones (GOT) to advance understanding of face blindness: Psychologists have used the TV series GOT to understand how the brain enables us to recognise faces. Their findings provide new insights into prosopagnosia or face blindness, a condition that impairs facial recognition.by /u/AnnaMouse247 on July 26, 2024 at 5:14 pm
submitted by /u/AnnaMouse247 [link] [comments]
- Specific genes may be related to the trajectory of recovery for stroke survivors, study finds. Researchers say genetic variants were strongly associated with depression, PTSD and cognitive health outcomes. Findings may provide useful insights for developing targeted therapies.by /u/AnnaMouse247 on July 26, 2024 at 5:08 pm
submitted by /u/AnnaMouse247 [link] [comments]
- New experimental drug shows promise in clearing HIV from brain: originally developed to treat cancer, study finds that by targeting infected cells in the brain, drug may clear virus from hidden areas that have been a major challenge in HIV treatment.by /u/AnnaMouse247 on July 26, 2024 at 4:57 pm
submitted by /u/AnnaMouse247 [link] [comments]
- Rapid diagnosis sepsis tests could decrease result wait times from days to hours, researchers report in Natureby /u/Science_News on July 26, 2024 at 3:50 pm
submitted by /u/Science_News [link] [comments]
Reddit Sports Sports News and Highlights from the NFL, NBA, NHL, MLB, MLS, and leagues around the world.
- Canada's men's and women's soccer teams have relied on drones and spying for years, sources sayby /u/FireLychee on July 26, 2024 at 3:37 am
submitted by /u/FireLychee [link] [comments]
- After a grueling Tour de France, top riders are racing to recover for Paris Olympics time trialby /u/Oldtimer_2 on July 26, 2024 at 12:54 am
submitted by /u/Oldtimer_2 [link] [comments]
- Canada WNT coach Bev Priestman suspended, sent home from Olympics amid spying scandalby /u/Oldtimer_2 on July 26, 2024 at 12:49 am
submitted by /u/Oldtimer_2 [link] [comments]
- 'End of an Era': TNT's 'Inside the NBA' Ending After NBA Chooses Amazonby /u/lame_building14 on July 26, 2024 at 12:36 am
submitted by /u/lame_building14 [link] [comments]
- Canada axes coach from Olympics over drone useby /u/chief_sitass on July 26, 2024 at 12:31 am
submitted by /u/chief_sitass [link] [comments]