AI Dashboard is available on the Web, Apple, Google, and Microsoft, PRO version
What is the Best Machine Learning Algorithms for Imbalanced Datasets?
In machine learning, imbalanced datasets are those where one class heavily outnumbers the others. This can be due to the nature of the problem or simply because more data is available for one class than the others. Either way, imbalanced datasets can pose a challenge for machine learning algorithms. In this blog post, we’ll take a look at which machine learning algorithms are best suited for imbalanced datasets and why they tend to perform better than others.
For example, in a binary classification problem, if there are 100 observations, and only 10 of them are positive (the rest are negatives), then we say that the dataset is imbalanced. The ratio of positive to negative cases is 1:10.

There are a few reasons why some machine learning algorithms tend to perform better on imbalanced datasets than others. First, certain algorithms are designed to handle imbalanced datasets. Second, some algorithms are more robust to outliers, which can be more common in imbalanced datasets. And third, some algorithms are better able to learn from a limited amount of data, which can be an issue when one class is heavily outnumbered by the others.
Some of the best machine learning algorithms for imbalanced datasets include:
– Support Vector Machines (SVMs),
– Decision Trees,
– Random Forests,
– Naive Bayes Classifiers,
– k-Nearest Neighbors (kNN),
Of these, SVMs tend to be the most popular choice as they are specifically designed to handle imbalanced datasets. SVMs work by finding a hyperplane that maximizes the margin between the two classes. This helps to reduce overfitting and improve generalization. Decision trees and random forests are also popular choices as they are less sensitive to outliers than other algorithms such as linear regression. Naive Bayes classifiers are another good choice as they are able to learn from a limited amount of data. kNN is also a good choice as it is not sensitive to outliers and is able to learn from a limited amount of data. However, it can be computationally intensive for large datasets.
There are two main types of machine learning algorithms: supervised and unsupervised. Supervised algorithms tend to perform better on imbalanced datasets than unsupervised algorithms. In this blog post, we will discuss why this is so and look at some examples.
Supervised Algorithms
Supervised algorithms are those where the target variable is known. In other words, we have training data where the correct answers are already given. The algorithm then learns from this data and is able to generalize to new data. Some examples of supervised algorithms are regression and classification.
Unsupervised Algorithms
Unsupervised algorithms are those where the target variable is not known. With unsupervised algorithms, we only have input data, without any corresponding output labels. The algorithm has to learn from the data itself without any guidance. Some examples of unsupervised algorithms are clustering and dimensionality reduction.
Why Supervised Algorithms Perform Better on Imbalanced Datasets
The reason why supervised algorithms perform better on imbalanced datasets is because they can learn from the training data which cases are more important. With unsupervised algorithms, all data points are treated equally, regardless of whether they are in the minority or majority class.
For example, in a binary classification problem with an imbalanced dataset, let’s say that we want to predict whether a customer will default on their loan payment or not. We have a training dataset of 1000 customers, out of which only 100 (10%) have defaulted on their loan in the past.
If we use a supervised algorithm like logistic regression, the algorithm will learn from the training data that defaulting on a loan is rare (since only 10% of cases in the training data are Positive). This means that it will be more likely to predict correctly that a new customer will not default on their loan (since this is the majority class in the training data).
However, if we use an unsupervised algorithm like k-means clustering, all data points will be treated equally since there is no target variable to guide the algorithm. This means that it might incorrectly cluster together customers who have defaulted on their loans with those who haven’t since there is no guidance provided by a target variable.
Conclusion:
In conclusion, supervised machine learning algorithms tend to perform better on imbalanced datasets than unsupervised machine learning algorithms because they can learn from the training data which cases are more important.
Some machine learning algorithms tend to perform better on highly imbalanced datasets because they are designed to deal with imbalance or because they can learn from both classes simultaneously. If you are working with a highly imbalanced dataset, then you should consider using one of these algorithms.
Thanks for reading!
How are machine learning techniques being used to address unstructured data challenges?
Machine learning techniques are being used to address unstructured data challenges in a number of ways:
- Natural language processing (NLP): NLP algorithms can be used to extract meaningful information from unstructured text data, such as emails, documents, and social media posts. NLP algorithms can be trained to classify text data, identify key terms and concepts, and extract structured data from unstructured text.
- Image recognition: Machine learning algorithms can be used to analyze and classify images, enabling the automatic identification and classification of objects, people, and other elements in images. This can be useful for tasks such as image tagging and search, as well as for applications such as security and surveillance.
- Audio and speech recognition: Machine learning algorithms can be used to analyze and classify audio data, enabling the automatic transcription and translation of spoken language. This can be useful for tasks such as speech-to-text transcription, as well as for applications such as call center automation and language translation.
- Video analysis: Machine learning algorithms can be used to analyze and classify video data, enabling the automatic detection and classification of objects, people, and other elements in video. This can be useful for tasks such as video tagging and search, as well as for applications such as security and surveillance.
Overall, machine learning techniques are being used in a wide range of applications to extract meaningful information from unstructured data, and to enable the automatic classification and analysis of data in a variety of formats.
How is AI and machine learning impacting application development today?
Artificial intelligence (AI) and machine learning are having a significant impact on application development today in a number of ways:
- Enabling new capabilities: AI and machine learning algorithms can be used to enable applications to perform tasks that would be difficult or impossible for humans to do. For example, AI-powered applications can be used to analyze and classify large amounts of data, or to automate complex decision-making processes.
- Improving performance: AI and machine learning algorithms can be used to optimize the performance of applications, making them faster, more efficient, and more accurate. For example, machine learning algorithms can be used to improve the accuracy of predictive models, or to optimize the performance of search algorithms.
- Streamlining development: AI and machine learning algorithms can be used to automate various aspects of application development, such as testing, debugging, and deployment. This can help to streamline the development process and reduce the time and resources needed to build and maintain applications.
- Enhancing user experiences: AI and machine learning algorithms can be used to enhance the user experience of applications, by providing personalized recommendations, recommendations, or by enabling applications to anticipate and respond to the needs and preferences of users.
Overall, AI and machine learning are having a significant impact on application development today, and they are likely to continue to shape the way applications are built and used in the future.
Get 20% off Google Google Workspace (Google Meet) Standard Plan with the following codes: 96DRHDRA9J7GTN6
Get 20% off Google Workspace (Google Meet) Business Plan (AMERICAS): M9HNXHX3WC9H7YE (Email us for more codes)
Active Anti-Aging Eye Gel, Reduces Dark Circles, Puffy Eyes, Crow's Feet and Fine Lines & Wrinkles, Packed with Hyaluronic Acid & Age Defying Botanicals
How will advancements in artificial intelligence and machine learning shape the future of work and society?
Advancements in artificial intelligence (AI) and machine learning are likely to shape the future of work and society in a number of ways. Some potential impacts include:
- Automation: AI and machine learning algorithms can be used to automate tasks that are currently performed by humans, such as data entry, customer service, and manufacturing. This could lead to changes in the types of jobs that are available and the skills that are in demand, as well as to increased productivity and efficiency.
- Job displacement: While automation may create new job opportunities, it could also lead to job displacement, particularly for workers in industries that are more susceptible to automation. This could lead to social and economic challenges, including unemployment and income inequality.
- Increased efficiency: AI and machine learning algorithms can be used to optimize and streamline business processes, leading to increased efficiency and productivity. This could lead to economic growth and innovation, and could also help to reduce costs for businesses and consumers.
- Enhanced decision-making: AI and machine learning algorithms can be used to analyze large amounts of data and make more informed and accurate decisions. This could lead to improved outcomes in fields such as healthcare, finance, and education, and could also help to reduce bias and improve fairness.
Overall, the impact of AI and machine learning on the future of work and society is likely to be significant and complex, with both potential benefits and challenges. It will be important to consider and address these impacts as these technologies continue to advance and become more widely adopted.
- [D] Modern best coding practices for Pytorch (for research)?by /u/SirBlobfish (Machine Learning) on May 1, 2024 at 9:24 pm
Hi all, I've been using Pytorch since 2019, and it has changed a lot in that time (especially since huggingface). Are there any modern guides/style-docs/example-repos you would recommend? For example, are namedtensors a good/common practice? Is Pytorch Lightning recommended? What are the best config management tools these days? How often do you use torch.script or torch.compile? submitted by /u/SirBlobfish [link] [comments]
- [D]Method to Address Residual Patterns in Forecast vs. Target Valuesby /u/tipoviento (Machine Learning) on May 1, 2024 at 8:12 pm
Hi everyone, I have a question about how to improve the XGBoost model performance when the residuals between forecasted values and the actual target values shows a pattern. In the graph I uploaded, each point represents an actual versus predicted pair. I've also added a diagonal line indicating a perfect match between predicted and actual values. What are some strategies I can explore to reduce this residual pattern and improve the alignment between my forecast and the actual values? Should I consider changing the model type, or are there other methods such as feature engineering or statistical adjustments that can address this issue? Any advice on model diagnostics or evaluation techniques that might help refine my model further? https://preview.redd.it/shj4g7m9hvxc1.png?width=846&format=png&auto=webp&s=b8e658c9ee7b8ce7612e3cd1f199a1682e056dca submitted by /u/tipoviento [link] [comments]
- [P] Lightweight Tool for Text to Image Segmentationby /u/Fun_Win_6054 (Machine Learning) on May 1, 2024 at 7:08 pm
Hi everyone, I'd like to introduce Switchify, a text prompt to image segmentation labelling tool. Check it out at https://runswitchify.com. Just sign up, upload an image, and start labelling. I think it'd be really useful for anyone trying to clean and process their image training data. I'd love any feedback on the product and general thoughts. Hope you guys enjoy trying it out. submitted by /u/Fun_Win_6054 [link] [comments]
- [R] Is Model Collapse Inevitable? Breaking the Curse of Recursion by Accumulating Real and Synthetic Databy /u/RSchaeffer (Machine Learning) on May 1, 2024 at 6:58 pm
submitted by /u/RSchaeffer [link] [comments]
- [R] Using Moving Average to train unsupervised LSTMby /u/RandomPasserBy44 (Machine Learning) on May 1, 2024 at 6:14 pm
Has anyone tried using Moving Average to train unsupervised LSTM for anomaly detection purposes or any ML? I have a dataset with date and value (essentially one feature). I was using a dataset with no anomalous data to train and hope it can reconstruct what normal trend should look like. However, while the val loss is low, the reconstruction was really bad and failed (constant line at 0). I was thinking if I should us Moving Average to smooth out the value because my dataset value actually jumps alot (however, it still only ranges between certain value, so is not an outlier) Does anyone have any tips on this? I'm just trying out a simple stacked LSTM. submitted by /u/RandomPasserBy44 [link] [comments]
- [P] I reproduced Anthropic's recent interpretability researchby /u/neverboosh (Machine Learning) on May 1, 2024 at 5:51 pm
Not that many people are paying attention to LLM interpretability research when capabilities research is moving as fast as it currently is, but interpretability is really important and in my opinion, really interesting and exciting! Anthropic has made a lot of breakthroughs in recent months, the biggest one being "Towards Monosemanticity". The basic idea is that they found a way to train a sparse autoencoder to generate interpretable features based on transformer activations. This allows us to look at the activations of a language model during inference, and understand which parts of the model are most responsible for predicting each next token. Something that really stood out to me was that the autoencoders they train to do this are actually very small, and would not require a lot of compute to get working. This gave me the idea to try to replicate the research by training models on my M3 Macbook. After a lot of reading and experimentation, I was able to get pretty strong results! I wrote a more in-depth post about it on my blog here: https://jakeward.substack.com/p/monosemanticity-at-home-my-attempt I'm now working on a few follow-up projects using this tech, as well as a minimal implementation that can run in a Colab notebook to make it more accessible. If you read my blog, I'd love to hear any feedback! submitted by /u/neverboosh [link] [comments]
- [R] KAN: Kolmogorov-Arnold Networksby /u/SeawaterFlows (Machine Learning) on May 1, 2024 at 5:03 pm
Paper: https://arxiv.org/abs/2404.19756 Code: https://github.com/KindXiaoming/pykan Quick intro: https://kindxiaoming.github.io/pykan/intro.html Documentation: https://kindxiaoming.github.io/pykan/ Abstract: Inspired by the Kolmogorov-Arnold representation theorem, we propose Kolmogorov-Arnold Networks (KANs) as promising alternatives to Multi-Layer Perceptrons (MLPs). While MLPs have fixed activation functions on nodes ("neurons"), KANs have learnable activation functions on edges ("weights"). KANs have no linear weights at all -- every weight parameter is replaced by a univariate function parametrized as a spline. We show that this seemingly simple change makes KANs outperform MLPs in terms of accuracy and interpretability. For accuracy, much smaller KANs can achieve comparable or better accuracy than much larger MLPs in data fitting and PDE solving. Theoretically and empirically, KANs possess faster neural scaling laws than MLPs. For interpretability, KANs can be intuitively visualized and can easily interact with human users. Through two examples in mathematics and physics, KANs are shown to be useful collaborators helping scientists (re)discover mathematical and physical laws. In summary, KANs are promising alternatives for MLPs, opening opportunities for further improving today's deep learning models which rely heavily on MLPs. https://preview.redd.it/r7vjmp31juxc1.png?width=2326&format=png&auto=webp&s=a2c722cf733510194659b9aaec24269a7f9e5d47 submitted by /u/SeawaterFlows [link] [comments]
- [D] Looking for a recent study/paper/article that showed that an alternate model with a similar number of parameters to a ViT performed just as well showning that there's nothing special about particular models.by /u/SunraysInTheStorm (Machine Learning) on May 1, 2024 at 5:00 pm
Title basically, this was a conversation I read just recently and am now looking for the source. A specific paper was mentioned in there as well. The conclusion drawn was that we might be at the limit of what we can do with statistical models and that there's nothing special about the models themselves - only the data that's fed matters. Any pointers would be appreciated, thanks! submitted by /u/SunraysInTheStorm [link] [comments]
- [p] AI powered SIEM/NIDSby /u/OpeningDirector1688 (Machine Learning) on May 1, 2024 at 4:50 pm
I know this might be a bit neiche for a subreddit like this but I’m just trying to get as much feedback as possible. I’m making a project. It was originally going to be a a type of AI powered SIEM. It would take in information from multiple network intrusion detection systems and detect patterns. E.g Suricata might flag a low level alert as well as SNORT and other simillar systems the same. Previously these alerts might have been disregarded but an AI powered SIEM could detect a pattern in each of these alerts, decide weather it’s an attack or just a false positive, and then decide what type of attack it is. Upon researching further into this project I have created an AI that can perform this task just from basic Netflow info. I’m aware this is a very broad question to ask but I was just wondering if anyone had any ideas for as the next step in this project. Maybe even a potential feature for the finished system. I’ve completed my original goal to a degree. If you have any questions feel free to ask. Any feedback would be amazing. Thanks for reading submitted by /u/OpeningDirector1688 [link] [comments]
- [2404.10667] VASA-1: Lifelike Audio-Driven Talking Faces Generated in Real Timeby /u/shadowylurking (Machine Learning) on May 1, 2024 at 4:05 pm
submitted by /u/shadowylurking [link] [comments]
- [D] ICML 2024 Paper Acceptance Resultby /u/zy415 (Machine Learning) on May 1, 2024 at 3:06 pm
ICML 2024 paper acceptance results are supposed to be soon. Creating a discussion thread for this year's results. There is so much noise in the reviews every year. Some good work that the authors are proud of might get rejected because of the noisy system, given that ICML is growing so large these years. We should keep in mind that the work is still valuable no matter what the final result is. submitted by /u/zy415 [link] [comments]
- [D] What does Speaker Embeddings consists of?by /u/Puzzleheaded_Bee5489 (Machine Learning) on May 1, 2024 at 1:42 pm
I'm working on a Speaker Verification project wherein I'm exploring different techniques to verify the speaker via voice. The traditional approach is to extract the MFCC, Filterbanks, and prosodic features. Now this method seems to be outdated as most of the research is focused on making use of pre-trained models like Nvidia's TitaNet, Microsoft's WavLM, SpeechBrain also a model for this. Now these pre-trained models give Embeddings as output which represent the speaker's voice regardless of what he said in the recording. Now my doubt is what do these Embeddings represent? One of the architecture's makes use of MFCC's and later passes them to NN like LSTM to capture the pattern. submitted by /u/Puzzleheaded_Bee5489 [link] [comments]
- What’s the current state of Multimodal LLM based Robotic Arm Strategy research ?[D][R]by /u/CrisYou (Machine Learning) on May 1, 2024 at 12:56 pm
I know like Figure Company’s robot used openai’s GPT as a brain and performs very well, able to do many chores already. So I wonder what spaces of robot ability left to improve?Any opinion is welcome! submitted by /u/CrisYou [link] [comments]
- [D] Is RPE still a valid approach, or is RoPE entirely superior?by /u/leoholt (Machine Learning) on May 1, 2024 at 12:37 pm
I'm working specifically on music (MIDI) generation with transformers. Most of these models/datasets are quite small, e.g. <100million parameters. If I understand correctly, RPE was quickly adapted with the Music Transformer as a means to embed the intra-token distance information into the attention calculations. Separately, RoPE seems to have a similar objective, although I can't grasp from the RoFormer paper if it embeds the same type (quality?) of knowledge (e.g. token X is ... distance from token Y). I guess my question is: for contexts where the models are small, and inter-token distance is crucially important, would RPE still potentially be a superior approach? submitted by /u/leoholt [link] [comments]
- [D] TensorDock — GPU Cloud Marketplace, H100s from $2.49/hrby /u/jonathan-lei (Machine Learning) on May 1, 2024 at 10:31 am
Hey folks! I’m Jonathan from TensorDock, and we’re building a cloud GPU marketplace. We want to make GPUs truly affordable and accessible. I once started a web hosting service on self-hosted servers in middle school. But building servers isn’t the same as selling cloud. There’s a lot of open source software to manage your homelab for side projects, but there isn’t anything to commercialize that. Large cloud providers charge obscene prices — so much so that they can often pay back their hardware in under 6 months with 24x7 utilization. We are building the software that allows anyone to become the cloud. We want to get to a point where any [insert company, data center, cloud provider with excess capacity] can install our software on our nodes and make money. They might not pay back their hardware in 6 months, but they don’t need to do the grunt work — we handle support, software, payments etc. In turn, you get to access a truly independent cloud: GPUs from around the world from suppliers who compete against each other on pricing and demonstrated reliability. So far, we’ve onboarded quite a few GPUs, including 200 NVIDIA H100 SXMs available from just $2.49/hr. But we also have A100 80Gs from $1.63/hr, A6000s from $0.47/hr, A4000s from $0.13/hr, etc etc. Because we are a true marketplace, prices fluctuate with supply and demand. All are available in plain Ubuntu 22.04 or with popular ML packages preinstalled — CUDA, PyTorch, TensorFlow, etc., and all are hosted by a network of mining farms, data centers, or businesses that we’ve closely vetted. If you’re looking for hosting for your next project, give us a try! Happy to provide testing credits, just email me at [jonathan@tensordock.com](mailto:jonathan@tensordock.com). And if you do end up trying us, please provide feedback below [or directly!] 🙂 Deploy a GPU VM: https://dashboard.tensordock.com/deploy CPU-only VMs: https://dashboard.tensordock.com/deploy_cpu Apply to become a host: https://tensordock.com/host submitted by /u/jonathan-lei [link] [comments]
- [D] How is max output length enforced in LLMs?by /u/Maltmax (Machine Learning) on May 1, 2024 at 9:25 am
Hi! I started pondering about how LLMs know when to stop generating tokens in response to a prompt. Is the notion of a stop token still used in modern LLMs? Or perhaps a combination of stop tokens and other tricks control the output length? From a fine tuning standpoint I get that you can train a model to always output tokens more or less the same length as in the training dataset. I.e. I imagine that the output length in instruction datasets are of similar length, and thus instruction fine tuned models learn to output the same length as in the dataset. If this is the case, then what about pre trained foundational models. Is the output length baked into the foundational model or only subsequent fine tuned models? submitted by /u/Maltmax [link] [comments]
- How does freezing a model work? [D]by /u/Small_Emotion8420 (Machine Learning) on May 1, 2024 at 7:24 am
In multimodal LLMs, they usually freeze a CLIP encoder. How does this work? Is it simply just a linear neuron, connecting the two inputs? Are there any papers/guides on this (specifically connecting 2 or more models together) submitted by /u/Small_Emotion8420 [link] [comments]
- [D] ICML 2024 Decision Threadby /u/hugotothechillz (Machine Learning) on May 1, 2024 at 7:01 am
ICML 2024 paper acceptance results are supposed to be released in 24 hours or so. I thought I might create this thread for us to discuss anything related to it. There is some noise in the reviews every year. Don’t forget that even though your paper might get rejected, this does not mean that it is not valuable work. Good luck everyone ! submitted by /u/hugotothechillz [link] [comments]
- Alice's Adventures in a Differentiable Wonderland -- Volume I, A Tour of the Landby /u/emiyake (Machine Learning) on May 1, 2024 at 2:21 am
submitted by /u/emiyake [link] [comments]
- [D] Lagrangian NN w Large Datasetby /u/CruisingLettuce (Machine Learning) on April 30, 2024 at 11:14 pm
I am trying to use a Lagrangian NN on a large medical dataset with 50+ features with one output col, A. How feasible is that? I feel like it loses some purpose in using LNN but am confident it may work. B. How do I get a Lagrangian NN to work on such a large dataset? It seems as though most LNNs I am seeing only have one input and one output col, but that would be next to impossible to implement with my dataset. Thanks submitted by /u/CruisingLettuce [link] [comments]
Active Hydrating Toner, Anti-Aging Replenishing Advanced Face Moisturizer, with Vitamins A, C, E & Natural Botanicals to Promote Skin Balance & Collagen Production, 6.7 Fl Oz
Age Defying 0.3% Retinol Serum, Anti-Aging Dark Spot Remover for Face, Fine Lines & Wrinkle Pore Minimizer, with Vitamin E & Natural Botanicals
Firming Moisturizer, Advanced Hydrating Facial Replenishing Cream, with Hyaluronic Acid, Resveratrol & Natural Botanicals to Restore Skin's Strength, Radiance, and Resilience, 1.75 Oz
Skin Stem Cell Serum
Smartphone 101 - Pick a smartphone for me - android or iOS - Apple iPhone or Samsung Galaxy or Huawei or Xaomi or Google Pixel
Can AI Really Predict Lottery Results? We Asked an Expert.
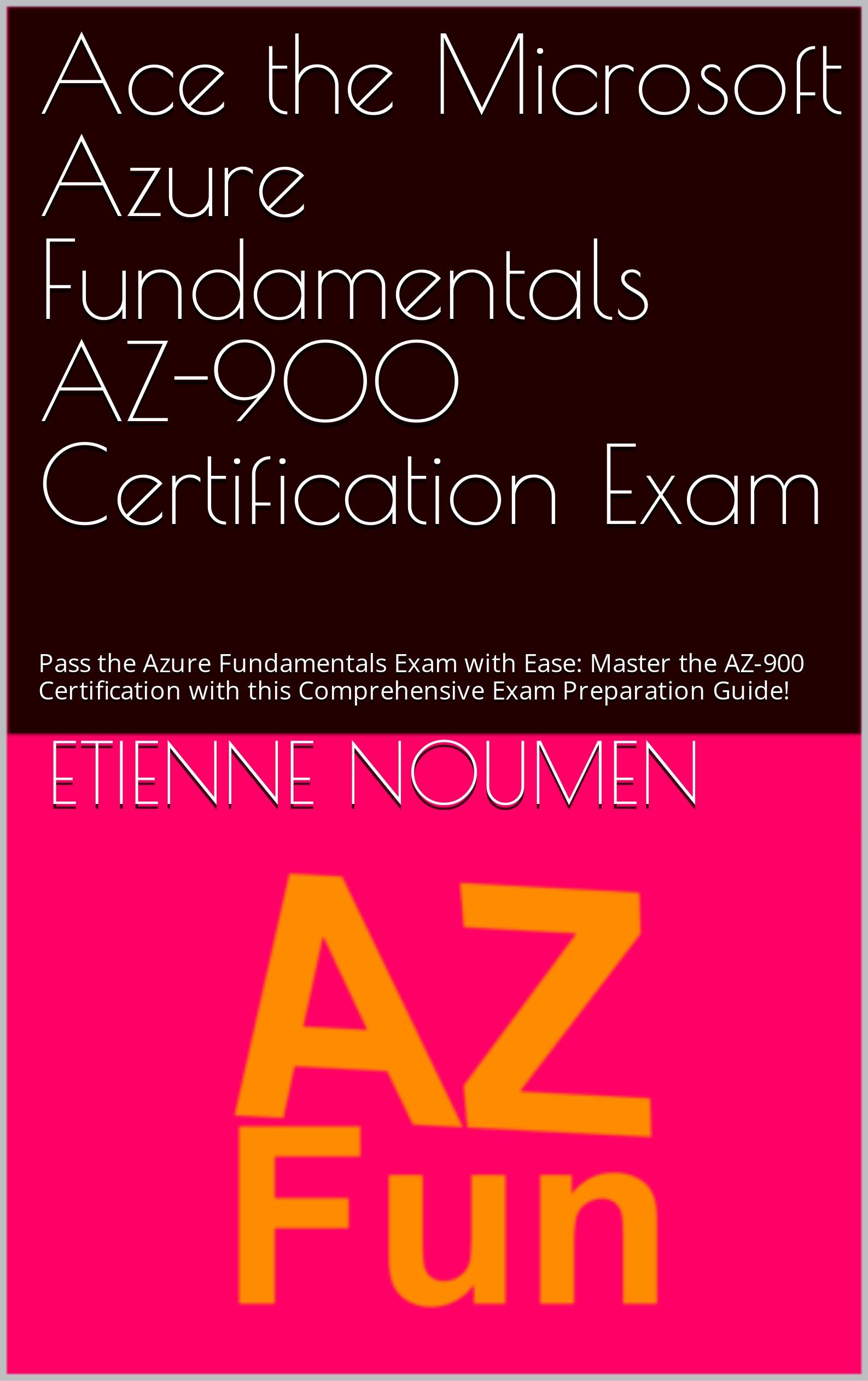

Djamgatech

Read Photos and PDFs Aloud for me iOS
Read Photos and PDFs Aloud for me android
Read Photos and PDFs Aloud For me Windows 10/11
Read Photos and PDFs Aloud For Amazon
Get 20% off Google Workspace (Google Meet) Business Plan (AMERICAS): M9HNXHX3WC9H7YE (Email us for more)
Get 20% off Google Google Workspace (Google Meet) Standard Plan with the following codes: 96DRHDRA9J7GTN6(Email us for more)
FREE 10000+ Quiz Trivia and and Brain Teasers for All Topics including Cloud Computing, General Knowledge, History, Television, Music, Art, Science, Movies, Films, US History, Soccer Football, World Cup, Data Science, Machine Learning, Geography, etc....

List of Freely available programming books - What is the single most influential book every Programmers should read
- Bjarne Stroustrup - The C++ Programming Language
- Brian W. Kernighan, Rob Pike - The Practice of Programming
- Donald Knuth - The Art of Computer Programming
- Ellen Ullman - Close to the Machine
- Ellis Horowitz - Fundamentals of Computer Algorithms
- Eric Raymond - The Art of Unix Programming
- Gerald M. Weinberg - The Psychology of Computer Programming
- James Gosling - The Java Programming Language
- Joel Spolsky - The Best Software Writing I
- Keith Curtis - After the Software Wars
- Richard M. Stallman - Free Software, Free Society
- Richard P. Gabriel - Patterns of Software
- Richard P. Gabriel - Innovation Happens Elsewhere
- Code Complete (2nd edition) by Steve McConnell
- The Pragmatic Programmer
- Structure and Interpretation of Computer Programs
- The C Programming Language by Kernighan and Ritchie
- Introduction to Algorithms by Cormen, Leiserson, Rivest & Stein
- Design Patterns by the Gang of Four
- Refactoring: Improving the Design of Existing Code
- The Mythical Man Month
- The Art of Computer Programming by Donald Knuth
- Compilers: Principles, Techniques and Tools by Alfred V. Aho, Ravi Sethi and Jeffrey D. Ullman
- Gödel, Escher, Bach by Douglas Hofstadter
- Clean Code: A Handbook of Agile Software Craftsmanship by Robert C. Martin
- Effective C++
- More Effective C++
- CODE by Charles Petzold
- Programming Pearls by Jon Bentley
- Working Effectively with Legacy Code by Michael C. Feathers
- Peopleware by Demarco and Lister
- Coders at Work by Peter Seibel
- Surely You're Joking, Mr. Feynman!
- Effective Java 2nd edition
- Patterns of Enterprise Application Architecture by Martin Fowler
- The Little Schemer
- The Seasoned Schemer
- Why's (Poignant) Guide to Ruby
- The Inmates Are Running The Asylum: Why High Tech Products Drive Us Crazy and How to Restore the Sanity
- The Art of Unix Programming
- Test-Driven Development: By Example by Kent Beck
- Practices of an Agile Developer
- Don't Make Me Think
- Agile Software Development, Principles, Patterns, and Practices by Robert C. Martin
- Domain Driven Designs by Eric Evans
- The Design of Everyday Things by Donald Norman
- Modern C++ Design by Andrei Alexandrescu
- Best Software Writing I by Joel Spolsky
- The Practice of Programming by Kernighan and Pike
- Pragmatic Thinking and Learning: Refactor Your Wetware by Andy Hunt
- Software Estimation: Demystifying the Black Art by Steve McConnel
- The Passionate Programmer (My Job Went To India) by Chad Fowler
- Hackers: Heroes of the Computer Revolution
- Algorithms + Data Structures = Programs
- Writing Solid Code
- JavaScript - The Good Parts
- Getting Real by 37 Signals
- Foundations of Programming by Karl Seguin
- Computer Graphics: Principles and Practice in C (2nd Edition)
- Thinking in Java by Bruce Eckel
- The Elements of Computing Systems
- Refactoring to Patterns by Joshua Kerievsky
- Modern Operating Systems by Andrew S. Tanenbaum
- The Annotated Turing
- Things That Make Us Smart by Donald Norman
- The Timeless Way of Building by Christopher Alexander
- The Deadline: A Novel About Project Management by Tom DeMarco
- The C++ Programming Language (3rd edition) by Stroustrup
- Patterns of Enterprise Application Architecture
- Computer Systems - A Programmer's Perspective
- Agile Principles, Patterns, and Practices in C# by Robert C. Martin
- Growing Object-Oriented Software, Guided by Tests
- Framework Design Guidelines by Brad Abrams
- Object Thinking by Dr. David West
- Advanced Programming in the UNIX Environment by W. Richard Stevens
- Hackers and Painters: Big Ideas from the Computer Age
- The Soul of a New Machine by Tracy Kidder
- CLR via C# by Jeffrey Richter
- The Timeless Way of Building by Christopher Alexander
- Design Patterns in C# by Steve Metsker
- Alice in Wonderland by Lewis Carol
- Zen and the Art of Motorcycle Maintenance by Robert M. Pirsig
- About Face - The Essentials of Interaction Design
- Here Comes Everybody: The Power of Organizing Without Organizations by Clay Shirky
- The Tao of Programming
- Computational Beauty of Nature
- Writing Solid Code by Steve Maguire
- Philip and Alex's Guide to Web Publishing
- Object-Oriented Analysis and Design with Applications by Grady Booch
- Effective Java by Joshua Bloch
- Computability by N. J. Cutland
- Masterminds of Programming
- The Tao Te Ching
- The Productive Programmer
- The Art of Deception by Kevin Mitnick
- The Career Programmer: Guerilla Tactics for an Imperfect World by Christopher Duncan
- Paradigms of Artificial Intelligence Programming: Case studies in Common Lisp
- Masters of Doom
- Pragmatic Unit Testing in C# with NUnit by Andy Hunt and Dave Thomas with Matt Hargett
- How To Solve It by George Polya
- The Alchemist by Paulo Coelho
- Smalltalk-80: The Language and its Implementation
- Writing Secure Code (2nd Edition) by Michael Howard
- Introduction to Functional Programming by Philip Wadler and Richard Bird
- No Bugs! by David Thielen
- Rework by Jason Freid and DHH
- JUnit in Action
#BlackOwned #BlackEntrepreneurs #BlackBuniness #AWSCertified #AWSCloudPractitioner #AWSCertification #AWSCLFC02 #CloudComputing #AWSStudyGuide #AWSTraining #AWSCareer #AWSExamPrep #AWSCommunity #AWSEducation #AWSBasics #AWSCertified #AWSMachineLearning #AWSCertification #AWSSpecialty #MachineLearning #AWSStudyGuide #CloudComputing #DataScience #AWSCertified #AWSSolutionsArchitect #AWSArchitectAssociate #AWSCertification #AWSStudyGuide #CloudComputing #AWSArchitecture #AWSTraining #AWSCareer #AWSExamPrep #AWSCommunity #AWSEducation #AzureFundamentals #AZ900 #MicrosoftAzure #ITCertification #CertificationPrep #StudyMaterials #TechLearning #MicrosoftCertified #AzureCertification #TechBooks
Top 1000 Canada Quiz and trivia: CANADA CITIZENSHIP TEST- HISTORY - GEOGRAPHY - GOVERNMENT- CULTURE - PEOPLE - LANGUAGES - TRAVEL - WILDLIFE - HOCKEY - TOURISM - SCENERIES - ARTS - DATA VISUALIZATION

Top 1000 Africa Quiz and trivia: HISTORY - GEOGRAPHY - WILDLIFE - CULTURE - PEOPLE - LANGUAGES - TRAVEL - TOURISM - SCENERIES - ARTS - DATA VISUALIZATION

Exploring the Pros and Cons of Visiting All Provinces and Territories in Canada.

Exploring the Advantages and Disadvantages of Visiting All 50 States in the USA

Health Health, a science-based community to discuss health news and the coronavirus (COVID-19) pandemic
- Potential negative effects of easing marijuana restrictionsby /u/Realistic-Plant3957 on May 1, 2024 at 3:55 pm
submitted by /u/Realistic-Plant3957 [link] [comments]
- Longest-ever COVID infection lasted more than 600 daysby /u/scientificamerican on May 1, 2024 at 2:36 pm
submitted by /u/scientificamerican [link] [comments]
- A Doctor at Cigna Said Her Bosses Pressured Her to Review Patients’ Cases Too Quickly. Cigna Threatened to Fire Her.by /u/Maxcactus on May 1, 2024 at 2:14 pm
submitted by /u/Maxcactus [link] [comments]
- USDA sampling ground beef in North Carolina, other states, to test for bird fluby /u/progress18 on May 1, 2024 at 12:48 pm
submitted by /u/progress18 [link] [comments]
- Rapidly rising levels of TFA ‘forever chemical’ alarm expertsby /u/app1etree on May 1, 2024 at 10:22 am
submitted by /u/app1etree [link] [comments]
Today I Learned (TIL) You learn something new every day; what did you learn today? Submit interesting and specific facts about something that you just found out here.
- TIL Underwater exploration was disallowed in Guanabara Bay by the Brazilian government after an Ancient Roman shipwreck was reportedly found that preceded Columbusby /u/BlueDragonizNotCool on May 1, 2024 at 2:46 pm
submitted by /u/BlueDragonizNotCool [link] [comments]
- TIL The African continent is being split in two by a 46 km (35 mile) long crack and will be two seperate land massess in a couple of million years.by /u/yeaheah on May 1, 2024 at 2:38 pm
submitted by /u/yeaheah [link] [comments]
- TIL about Cougar Annie, who "birthed 11 children, outlived four husbands and became known for allegedly shooting and killing about 70 cougars in her lifetime" after moving to the west coast of Vancouver Island.by /u/MaximinusRats on May 1, 2024 at 2:12 pm
submitted by /u/MaximinusRats [link] [comments]
- TIL not only does it snow in the Sahara desert, but snowfall in Sahara Desert is getting more frequent!by /u/ThisCarSmellsFunny on May 1, 2024 at 12:43 pm
submitted by /u/ThisCarSmellsFunny [link] [comments]
- TIL in 1998 Lay's introduced fat free "WOW" chips containing a fat substitute called "Olestra." They were incredibly popular with $400 million in sales their first year. The following year sales dropped in half as Olestra caused side effects like "abdominal cramping, diarrhea, and "anal leakage"by /u/BDWG4EVA on May 1, 2024 at 11:00 am
submitted by /u/BDWG4EVA [link] [comments]
Reddit Science This community is a place to share and discuss new scientific research. Read about the latest advances in astronomy, biology, medicine, physics, social science, and more. Find and submit new publications and popular science coverage of current research.
- Throwing cold water on muscle growth: A systematic review with meta-analysis of the effects of postexercise cold water immersion on resistance training-induced hypertrophyby /u/inde_ on May 1, 2024 at 3:32 pm
submitted by /u/inde_ [link] [comments]
- What Makes a Society More Resilient | Frequent Hardship. (Gift Article) | "frequent disturbances enhance a population’s capacity to resist and recover from later downturns"by /u/mem_somerville on May 1, 2024 at 3:31 pm
submitted by /u/mem_somerville [link] [comments]
- New mRNA cancer vaccine technique using “onion-like” multi-lamellar RNA lipid particle aggregates shows success in treating brain cancer in 4 humansby /u/globehater on May 1, 2024 at 3:08 pm
submitted by /u/globehater [link] [comments]
- Loneliness in adulthood follows a U-shaped pattern: it's higher in younger and older adulthood, and lowest during middle adulthood, reports a new study that examined nine longitudinal studies from around the world.by /u/Wagamaga on May 1, 2024 at 2:26 pm
submitted by /u/Wagamaga [link] [comments]
- Microbiome confounders and quantitative profiling challenge predicted microbial targets in colorectal cancer developmentby /u/SaltZookeepergame691 on May 1, 2024 at 2:05 pm
submitted by /u/SaltZookeepergame691 [link] [comments]
Reddit Sports Sports News and Highlights from the NFL, NBA, NHL, MLB, MLS, and leagues around the world.
- Bill Romanowski files for bankruptcy as government seeks $15.5 million in back taxesby /u/Oldtimer_2 on May 1, 2024 at 3:28 pm
submitted by /u/Oldtimer_2 [link] [comments]
- Videos of Georgia pitcher before and during his dominant outing leads A&M coach to suspect cheatingby /u/Oldtimer_2 on May 1, 2024 at 3:19 pm
submitted by /u/Oldtimer_2 [link] [comments]
- Tennis legend Boris Becker discharged from bankruptcy court in Englandby /u/Oldtimer_2 on May 1, 2024 at 2:09 pm
submitted by /u/Oldtimer_2 [link] [comments]
- Rockies become first to team to trail in opening 29 games, waste 5-run lead in 7-6 loss to Marlinsby /u/Oldtimer_2 on May 1, 2024 at 2:06 pm
submitted by /u/Oldtimer_2 [link] [comments]
- U.S. Rowing report finds abuse allegations vs. Ted Nash credibleby /u/PrincessBananas85 on May 1, 2024 at 11:37 am
submitted by /u/PrincessBananas85 [link] [comments]